BridgeNet: A Joint Learning Network of Depth Map
Super-Resolution and Monocular Depth Estimation
2 UISEE, Beijing, China
3 City University of Hong Kong, China
Abstract
Depth map super-resolution is a task with high practical application requirements in the industry. Existing color-guided depth map super-resolution methods usually necessitate an extra branch to extract high-frequency detail information from RGB image to guide the low-resolution depth map reconstruction. However, because there are still some differences between the two modalities, direct information transmission in the feature dimension or edge map dimension cannot achieve satisfactory result, and may even trigger texture copying in areas where the structures of the RGB-D pair are inconsistent. Inspired by the multi-task learning, we propose a joint learning network of depth map super-resolution (DSR) and monocular depth estimation (MDE) without introducing additional supervision labels. For the interaction of two subnetworks, we adopt a differentiated guidance strategy and design two bridges correspondingly. One is the high-frequency attention bridge (HABdg) designed for the feature encoding process, which learns the high-frequency information of the MDE task to guide the DSR task. The other is the content guidance bridge (CGBdg) designed for the depth map reconstruction process, which provides the content guidance learned from DSR task for MDE task. The entire network architecture is highly portable and can provide a paradigm for associating the DSR and MDE tasks. Extensive experiments on benchmark datasets demonstrate that our method achieves competitive performance.
Pipeline
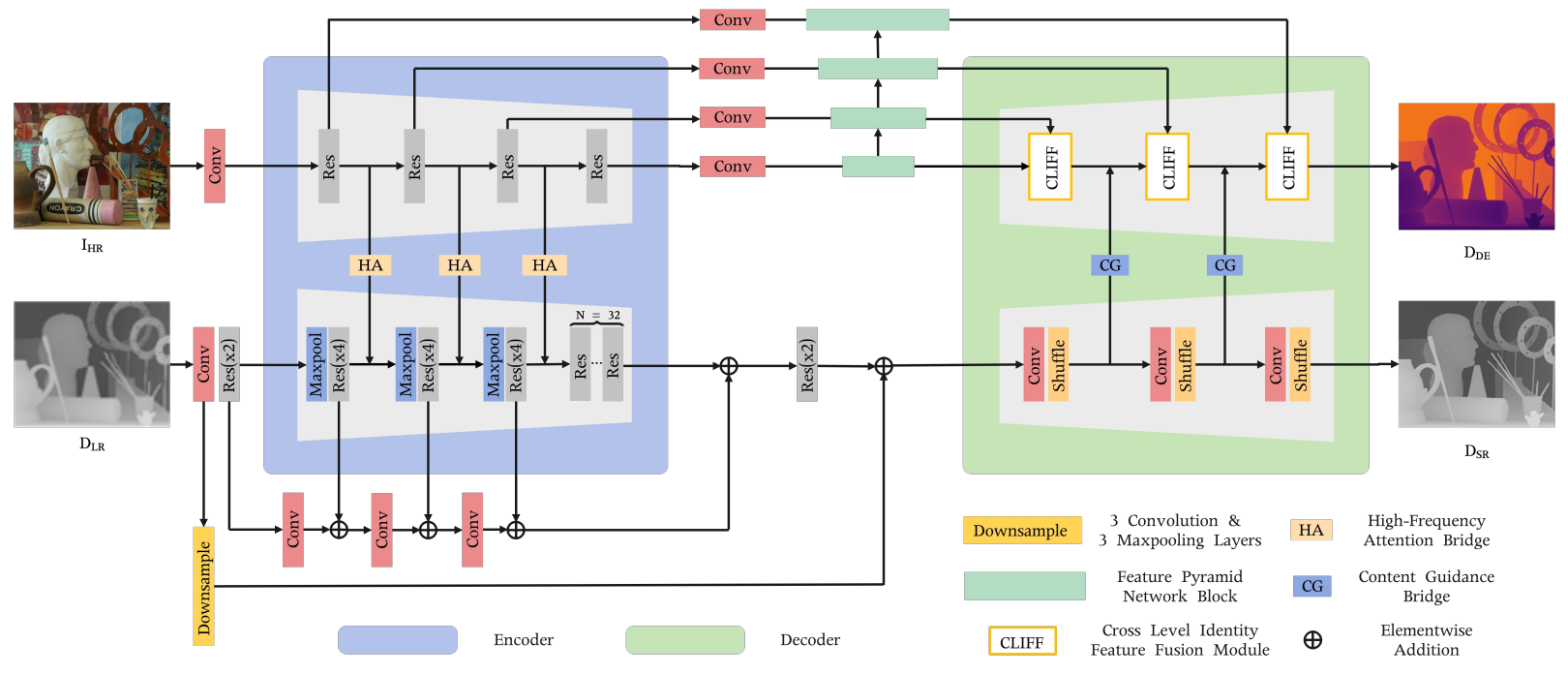
Architecture of BridgeNet, , which consists of a depth super-resolution subnetwork (DSRNet), a monocular depth estimation subnetwork (MDENet), a high-frequency attention bridge (HABdg), and a content guidance bridge (CGBdg). The encoder-decoder structure at the top is the MDENet, and the bottom encoder-decoder structure corresponds to the DSRNet. The HABdg works between the feature encoders of the two subnetworks, focusing on passing the high-frequency color guidance obtained from MDENet to DSRNet. On the contrary, CGBdg works on the decoder side and is used to provide the MDENet with content guidance information learned from the DSRNet.
Highlights
This work attempts to associate the depth map super-resolution and monocular depth estimation in a joint learning framework to boost the performance of depth map super-resolution, including a depth super-resolution network (DSRNet), a monocular depth estimation network (MDENet), and two joint learning bridges. Our entire network architecture is highly portable and can provide a paradigm for associating the DSR and MDE tasks. Moreover, different from other multi-task learning, no additional labels are required.
The high-frequency attention bridge (HABdg) in the feature encoding stage transfers the RGB high-frequency information learned from MDENet to DSRNet, which can provide color guidance information closer to the depth modality. Following the principle of simple task guiding difficult task, we switch the guiding roles of the two tasks in the feature decoding stage, and propose the content guidance bridge (CGBdg) to provide the content guidance learned from DSRNet for MDENet.
Without the introduction of additional supervision labels, our method achieves competitive performance on benchmark datasets.
Qualitative Evaluation
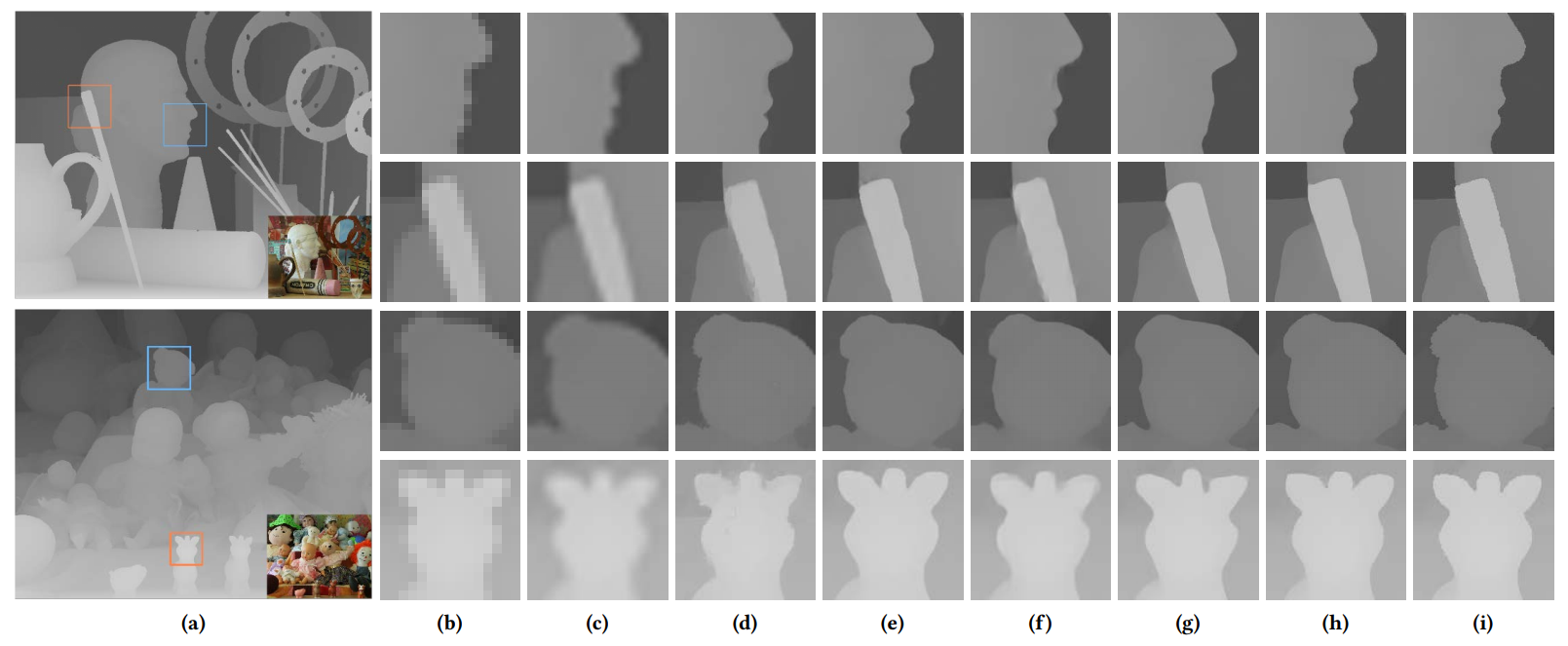
Visual comparisons of X8 up-sampling results on two examples (i.e., Art in the first row and Dolls in the second row). (a) Ground truth depth maps and color images; (b) LR depth patches; (c)-(h) The super-resolved depth maps generated by Bicubic, TGV, MSG, DGDIE, CTKT, and BridgeNet, respectively. (i) Ground truth. Depth patches are enlarged for clear visualization.
Quantitative Evaluation
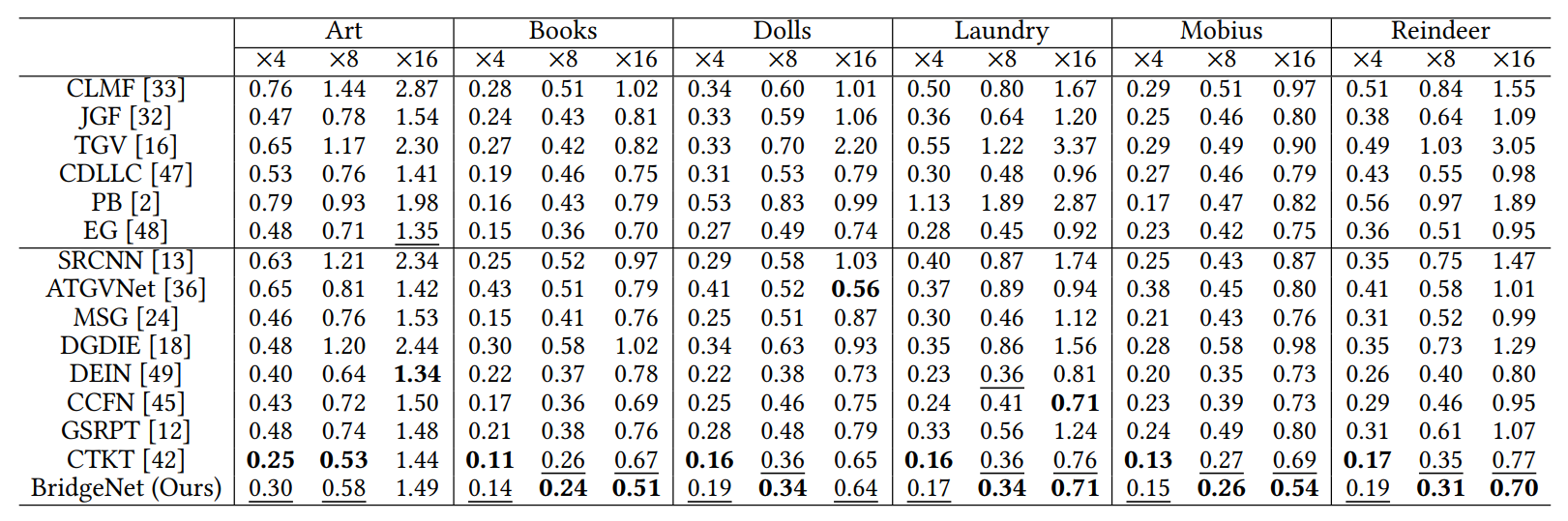
Quantitative depth SR results (in MAD) on Middlebury 2005 dataset. The best performance is displayed in bold, and the second best performance is marked in underline.
Citation
@inproceedings{BridgeNet, title={{BridgeNet}: A Joint Learning Network of Depth Map Super-Resolution and Monocular Depth Estimation}, author={Tang, Qi and Cong, Runmin and Sheng, Ronghui and He, Lingzhi and Zhang, Dan and Zhao, Yao and Kwong, Sam}, booktitle={Proc. ACM MM}, year={2021} }
Contact
If you have any questions, please contact Runmin Cong at rmcong@bjtu.edu.cn.