Cross-modality Discrepant Interaction Network
for RGB-D Salient Object Detection
2 Meituan, Beijing, China
3 City University of Hong Kong, China
Abstract
The popularity and promotion of depth maps have brought new vigor and vitality into salient object detection (SOD), and a mass of RGB-D SOD algorithms have been proposed, mainly concentrating on how to better integrate cross-modality features from RGB image and depth map. For the cross-modality interaction in feature encoder, existing methods either indiscriminately treat RGB and depth modalities, or only habitually utilize depth cues as auxiliary information of the RGB branch. Different from them, we reconsider the status of two modalities and propose a novel Cross-modality Discrepant Interaction Network (CDINet) for RGB-D SOD, which differentially models the dependence of two modalities according to the feature representations of different layers. To this end, two components are designed to implement the effective cross-modality interaction: 1) the RGB-induced Detail Enhancement (RDE) module leverages RGB modality to enhance the details of the depth features in low-level encoder stage. 2) the Depth-induced Semantic Enhancement (DSE) module transfers the object positioning and internal consistency of depth features to the RGB branch in high-level encoder stage. Furthermore, we also design a Dense Decoding Reconstruction (DDR) structure, which constructs a semantic block by combining multi-level encoder features to upgrade the skip connection in the feature decoding. Extensive experiments on five benchmark datasets demonstrate that our network outperforms 15 state-of-the-art methods both quantitatively and qualitatively.
Movations
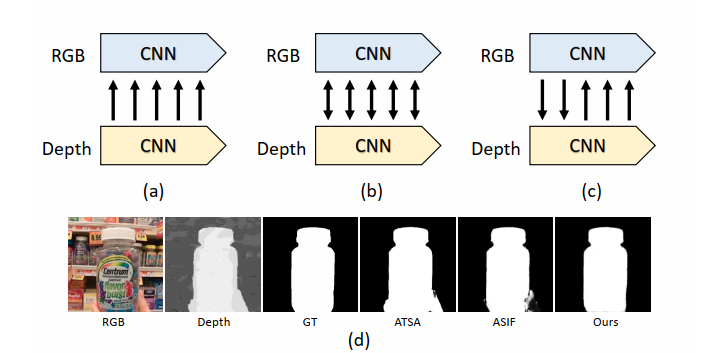
Previous studies have confirmed the positive effect of RGB and depth information interaction in SOD tasks. In this paper, we seriously reconsider the status of RGB modality and depth modality in RGB-D SOD task. Different from the previous unidirectional interaction shown in Figure (a) and undifferentiated bidirectional interaction shown in Figure (b), we believe that the interaction of the two modalities information should be carried out in a separate and discrepant manner. The low-level RGB features can help the depth features to distinguish different object instances at the same depth level, while the high-level depth features can further enrich the RGB semantics and suppress background interference. Therefore, a perfect RGB-D SOD model should give full play to the advantages of each modality, and simultaneously utilize another modality to make up for itself to avoid causing interference. To this end, we propose a cross-modality discrepant interaction network to explicitly model the dependence of two modalities in the encoder stage according to the feature representations of different layers, which selectively utilizes RGB features to supplement the details for depth branch, and transfers the depth features to RGB modality to enrich the semantic representations.
Pipeline
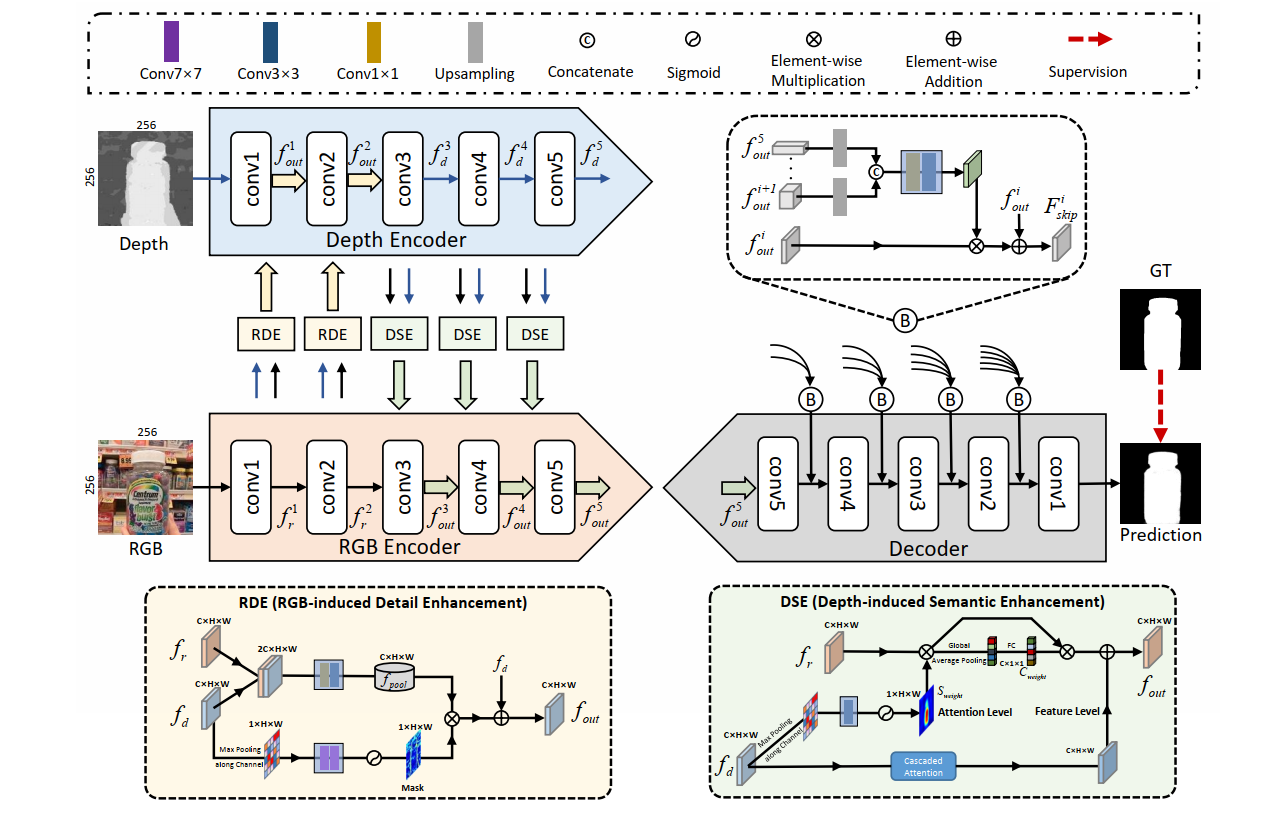
The overall pipeline of the proposed CDINet. Our CDINet follows an encoder-decoder architecture, which realizes the discrepant interaction and guidance of cross-modality information in the encoding stage. The framework mainly consists of three parts: 1) RGB-induced detail enhancement module. It achieves depth feature enhancement by transmitting the detailed supplementary information of the RGB modality to the depth modality. 2) Depth-induced semantic enhancement module. Depth features provide better positioning and internal consistency to enrich the semantic information of RGB features. 3) Dense decoding reconstruction structure. It densely encodes the encoder features of different layers to generate more valuable skip connection information, which is shown in the top right box marked B of this figure. The backbone of our network in this figure is VGG16, and the overall network can be trained efficiently as an end-to-end system.
Highlights
We propose an end-to-end Cross-modality Discrepant Interaction Network (CDINet), which differentially models the dependence of two modalities according to the feature representations of different layers. Our network achieves competitive performance against 15 state-of-the-art methods on 5 RGB-D SOD datasets. Moreover, the inference speed for an image reaches 42 FPS.
We design an RGB-induced Detail Enhancement (RDE) module to transfer detail supplement information from RGB modality to depth modality in low-level encoder stage, and a Depth-induced Semantic Enhancement (DSE) module to assist RGB branch in capturing clearer and fine-grained semantic attributes by utilizing the advantage of positioning accuracy and internal consistency of high-level depth features.
We design a Dense Decoding Reconstruction (DDR) structure, which generates a semantic block by leveraging multiple high-level encoder features to upgrade the skip connection in the feature decoding.
Qualitative Evaluation
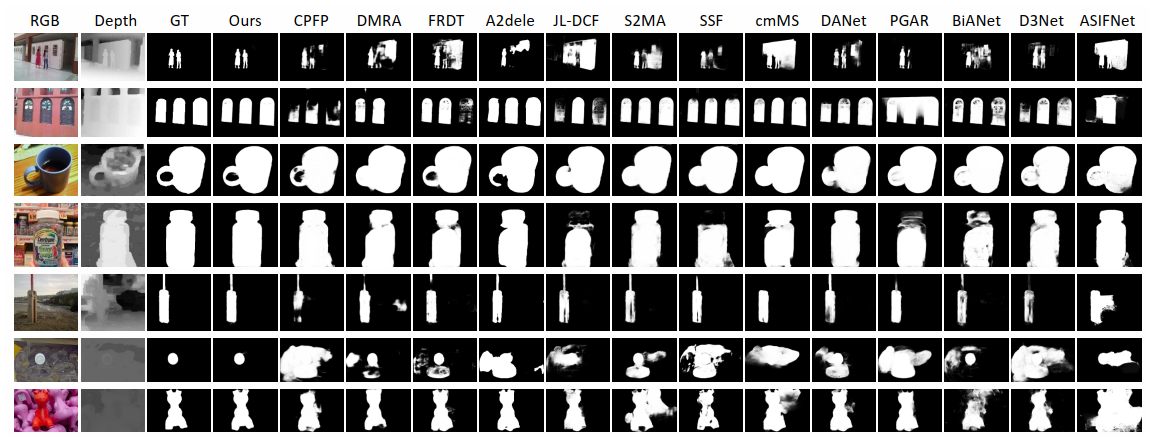
Quantitative Evaluation
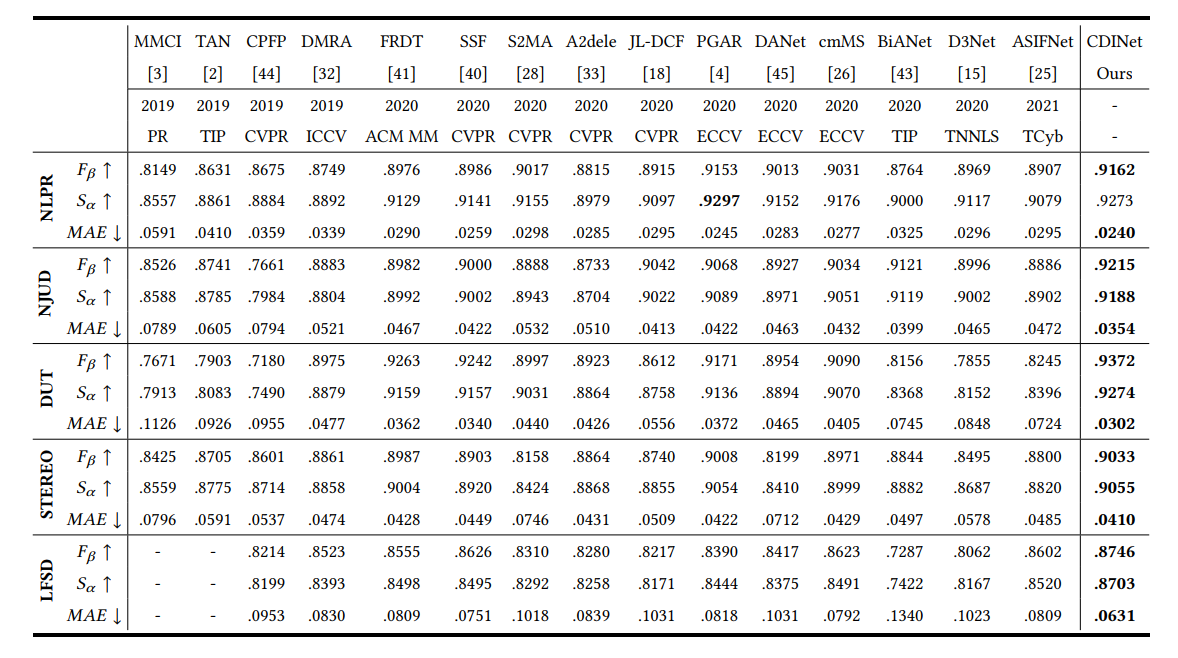
Citation
@inproceedings{CDINet, title={Cross-modality Discrepant Interaction Network for {RGB-D} Salient Object Detection}, author={Zhang, Chen and Cong, Runmin and Lin, Qinwei and Ma, Lin and Li, Feng and Zhao, Yao and Kwong, Sam}, booktitle={Proc. ACM MM}, pages={2094-2102}, year={2021} }
Contact
If you have any questions, please contact Runmin Cong at rmcong@bjtu.edu.cn.