CIR-Net: Cross-modality Interaction and Refinement for RGB-D Salient Object Detection
2 Nanyang Technological University, Singapore
3 Sun Yat-sen University, Shenzhen, China
4University of Chinese Academy of Sciences, Beijing, China
Abstract
Focusing on the issue of how to effectively capture and utilize cross-modality information in RGB-D salient object detection (SOD) task, we present a convolutional neural network (CNN) model, named CIR-Net, based on the novel cross-modality interaction and refinement. For the cross-modality interaction, 1) a progressive attention guided integration unit is proposed to sufficiently integrate RGB-D feature representations in the encoder stage, and 2) a convergence aggregation structure is proposed, which flows the RGB and depth decoding features into the corresponding RGB-D decoding streams via an importance gated fusion unit in the decoder stage. For the cross-modality refinement, we insert a refinement middleware structure between the encoder and the decoder, in which the RGB, depth, and RGB-D encoder features are further refined by successively using a self-modality attention refinement unit and a cross-modality weighting refinement unit. At last, with the gradually refined features, we predict the saliency map in the decoder stage. Extensive experiments on six popular RGB-D SOD benchmarks demonstrate that our network outperforms the state-of-the-art saliency detectors both qualitatively and quantitatively.
Pipeline
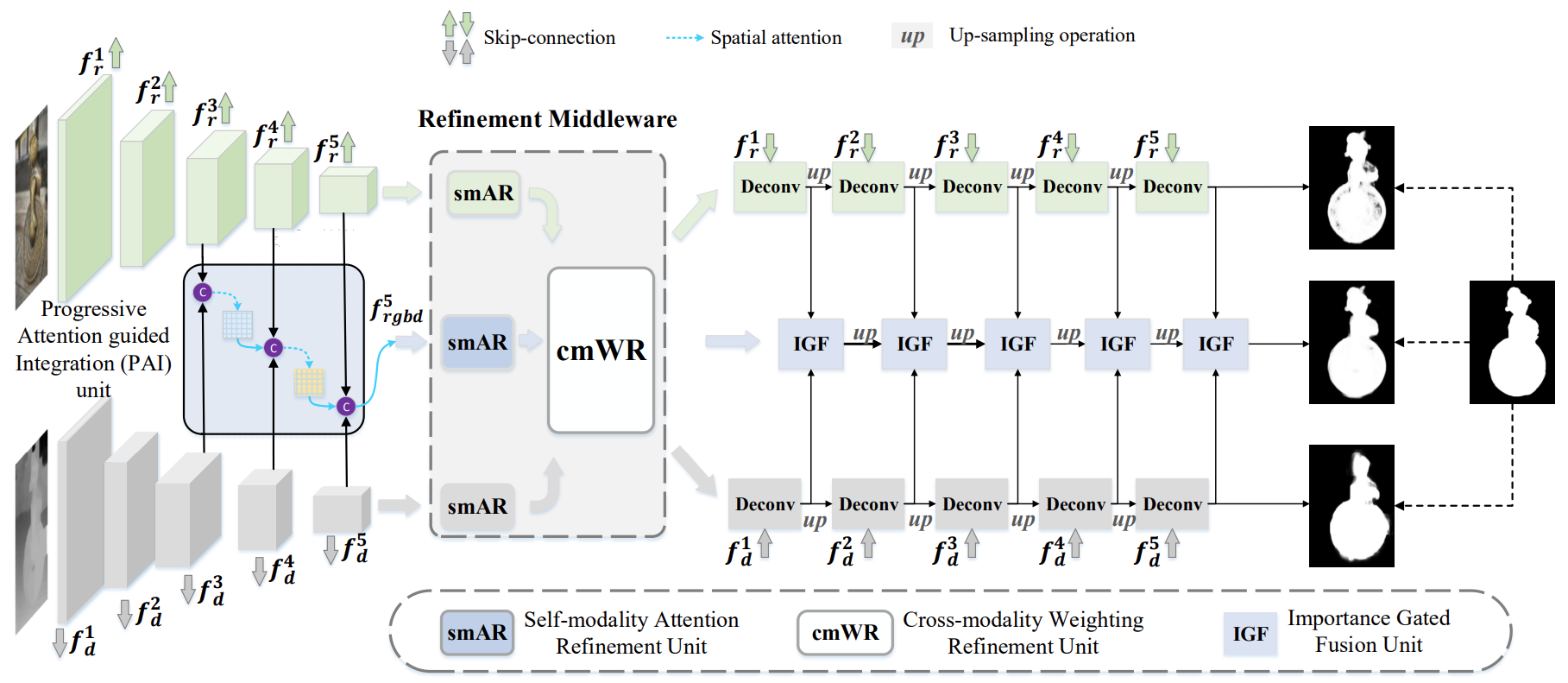
The overview of the proposed CIR-Net. The extracted RGB and depth features from the backbone. In the feature encoder, we also use the PAI unit to generate the cross-modality RGB-D encoder features. Then, the top-layer RGB, depth, and RGB-D features are embedded into the refinement middleware consisting of a smAR unit and a cmWR unit to progressively refine the multi-modality encoder features in a self- and cross-modality manner. Finally, the decoder features of the RGB branch and depth branch flow into the corresponding RGB-D stream to learn more comprehensive interaction features through an IGF unit in the feature decoder stage. Note that all three branches output a corresponding saliency prediction map, and we use the output of the RGB-D branch as the final result.
Model Details
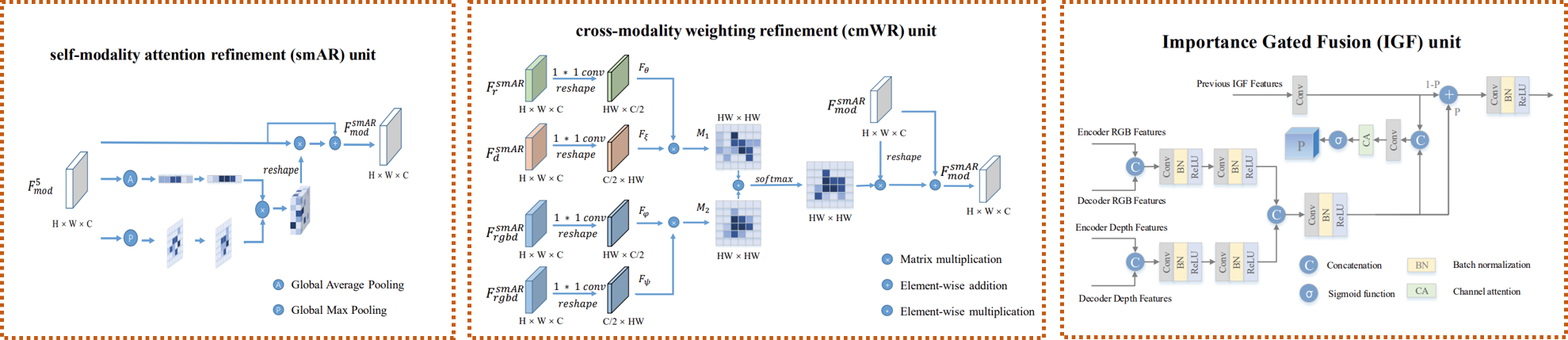
Illustration of the self-modality attention refinement (smAR) unit and the cross-modality weighting refinement (cmWR) unit in the refinement middleware structure, and the importance gated fusion (IGF) unit.
Highlights
We propose an end-to-end cross-modality interaction and refinement network (CIR-Net) for RGB-D SOD by fully capturing and utilizing the cross-modality information in an interaction and refinement manner.
The progressive attention guided integration unit and the importance gated fusion unit are proposed to achieve comprehensive cross-modality interaction in the encoder and decoder stages respectively.
The refinement middleware structure including the self-modality attention refinement unit and cross-modality weighting refinement unit is designed to refine the multi-modality encoder features by encoding the self-modality 3D attention tensor and the cross-modality contextual dependencies.
Without any pre-processing (e.g., HHA) or post-processing (e.g., CRF) techniques, our network achieves competitive performance against the state-of-the-art methods on six RGB-D SOD datasets.
Qualitative Evaluation
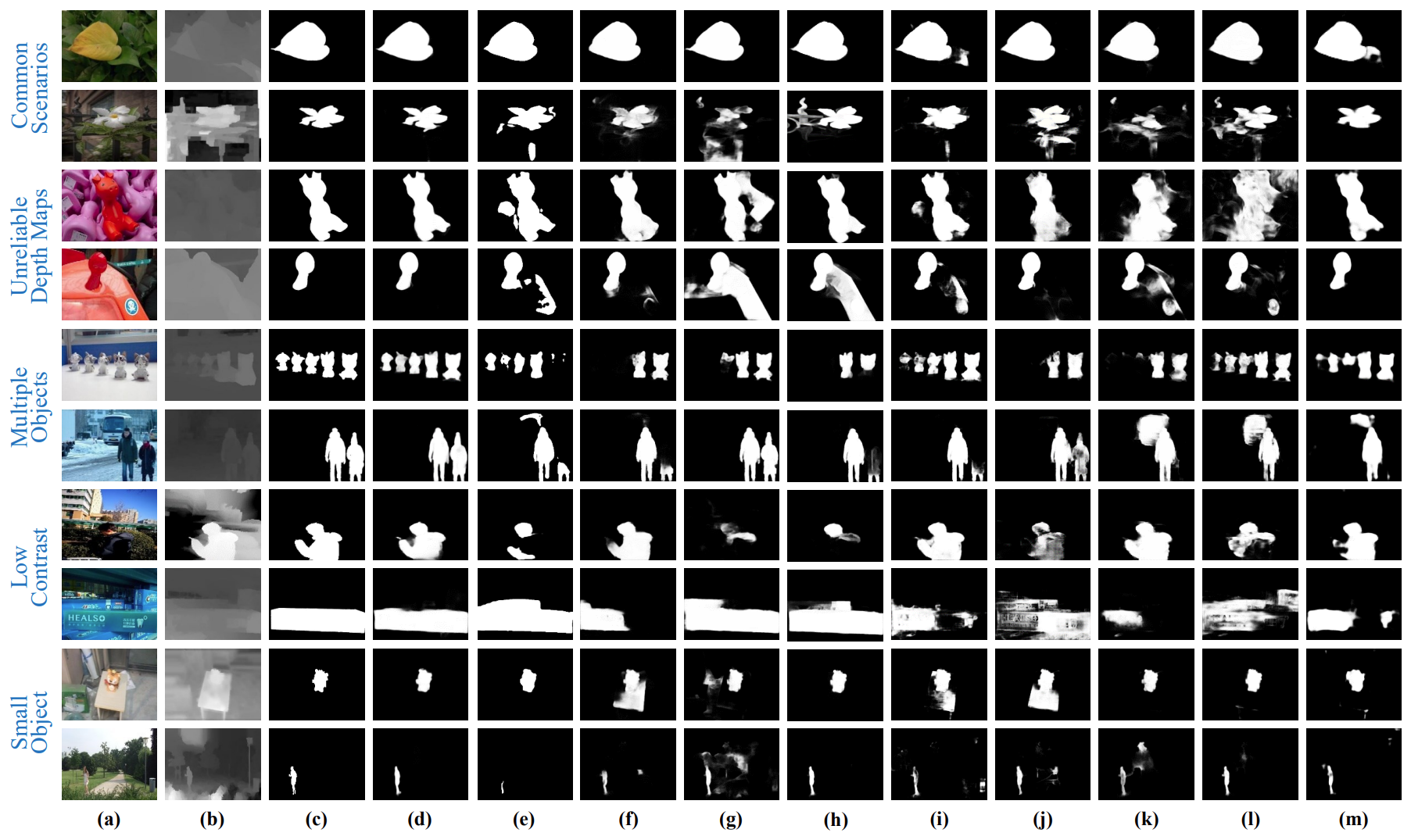
Visual examples of different methods. (a) RGB image. (b) Depth map. (c) GT. (d) Ours. (e) A2dele. (f) DANet. (g) S2MA. (h) PGAR. (i) FRDT. (j) JL-DCF. (k) D3Net. (l) BiANet. (m) DMRA. Our method outperforms other SOTA algorithms in various scenes, including common scenarios (line 1 and 2), unreliable or confusing depth maps (line 3 and 4), multiple objects (line 5 and 6), low contrast (line 7 and 8), and small objects (line 9 and 10).
Quantitative Evaluation
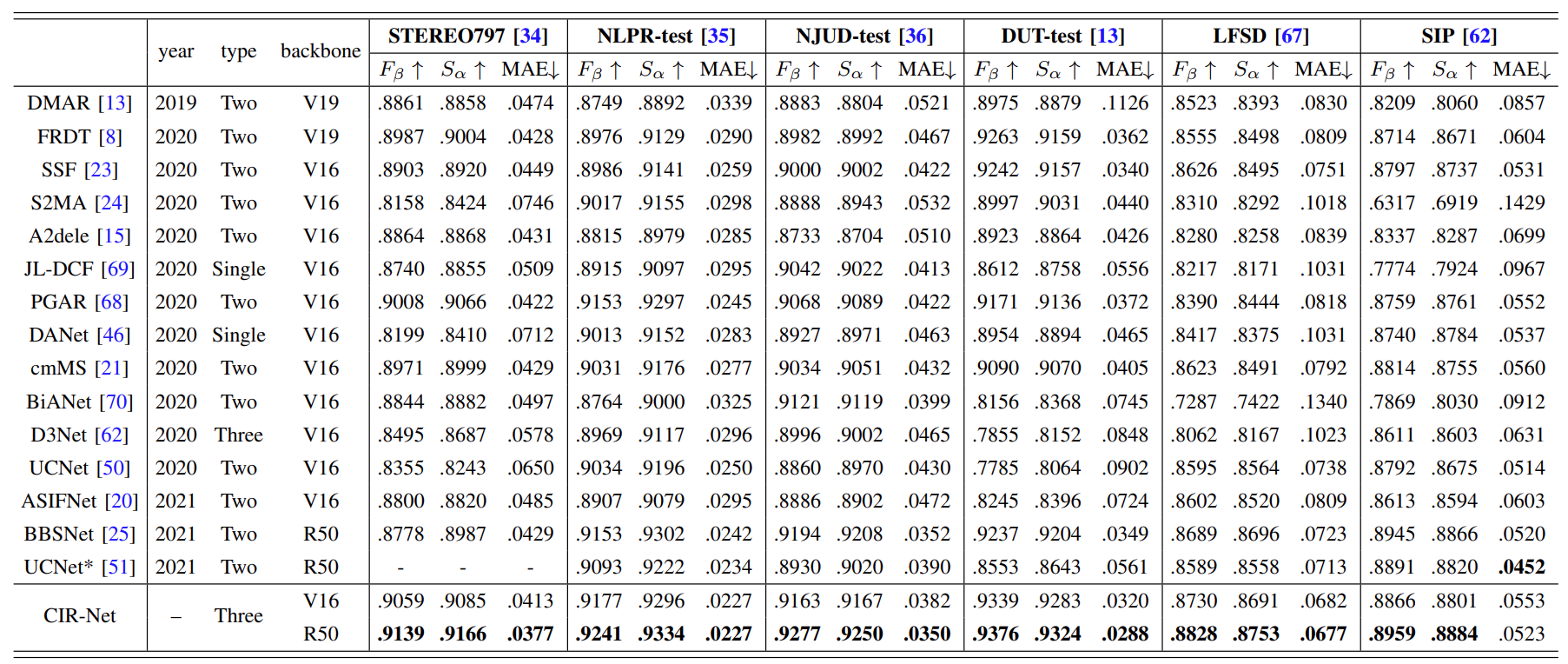
Quantitative comparison resutls in terms of S-measure, maximum F-measure, and MAE on six RGB-D benchmark datasets. The bold indicates the best result under each case. The type indicates whether the method is single-stream, two-stream, or three-stream. V16, V19 and R50 denote VGG16, VGG19 and ResNet50, respectively.
Citation
@article{CIRNet, title={{CIR-Net}: Cross-modality interaction and refinement for {RGB-D} salient object detection}, author={Cong, Runmin and Lin, Qinwei and Zhang, Chen and Li, chongyi and Cao, Xiaochun and Huang, Qingming and Zhao, Yao }, journal={IEEE Transactions on Image Processing}, volume={31}, pages={6800-6815}, year={2022}, publisher={IEEE} }
Contact
If you have any questions, please contact Runmin Cong at rmcong@bjtu.edu.cn.