CoADNet: Collaborative Aggregation-and-Distribution Networks for Co-Salient Object Detection
2 Beijing Jiaotong University, Beijing, China
3 Nanyang Technological University, Singapore
Abstract
Co-Salient Object Detection (CoSOD) aims at discovering salient objects that repeatedly appear in a given query group containing two or more relevant images. One challenging issue is how to effectively capture co-saliency cues by modeling and exploiting inter-image relationships. In this paper, we present an end-to-end collaborative aggregation-and-distribution network (CoADNet) to capture both salient and repetitive visual patterns from multiple images. First, we integrate saliency priors into the backbone features to suppress the redundant background information through an online intra-saliency guidance structure. After that, we design a two-stage aggregate-and-distribute architecture to explore group-wise semantic interactions and produce the co-saliency features. In the first stage, we propose a group-attentional semantic aggregation module that models inter-image relationships to generate the group-wise semantic representations. In the second stage, we propose a gated group distribution module that adaptively distributes the learned group semantics to different individuals in a dynamic gating mechanism. Finally, we develop a group consistency preserving decoder tailored for the CoSOD task, which maintains group constraints during feature decoding to predict more consistent full-resolution co-saliency maps. The proposed CoADNet is evaluated on four prevailing CoSOD benchmark datasets, which demonstrates the remarkable performance improvement over ten state-of-the-art competitors.
Pipeline
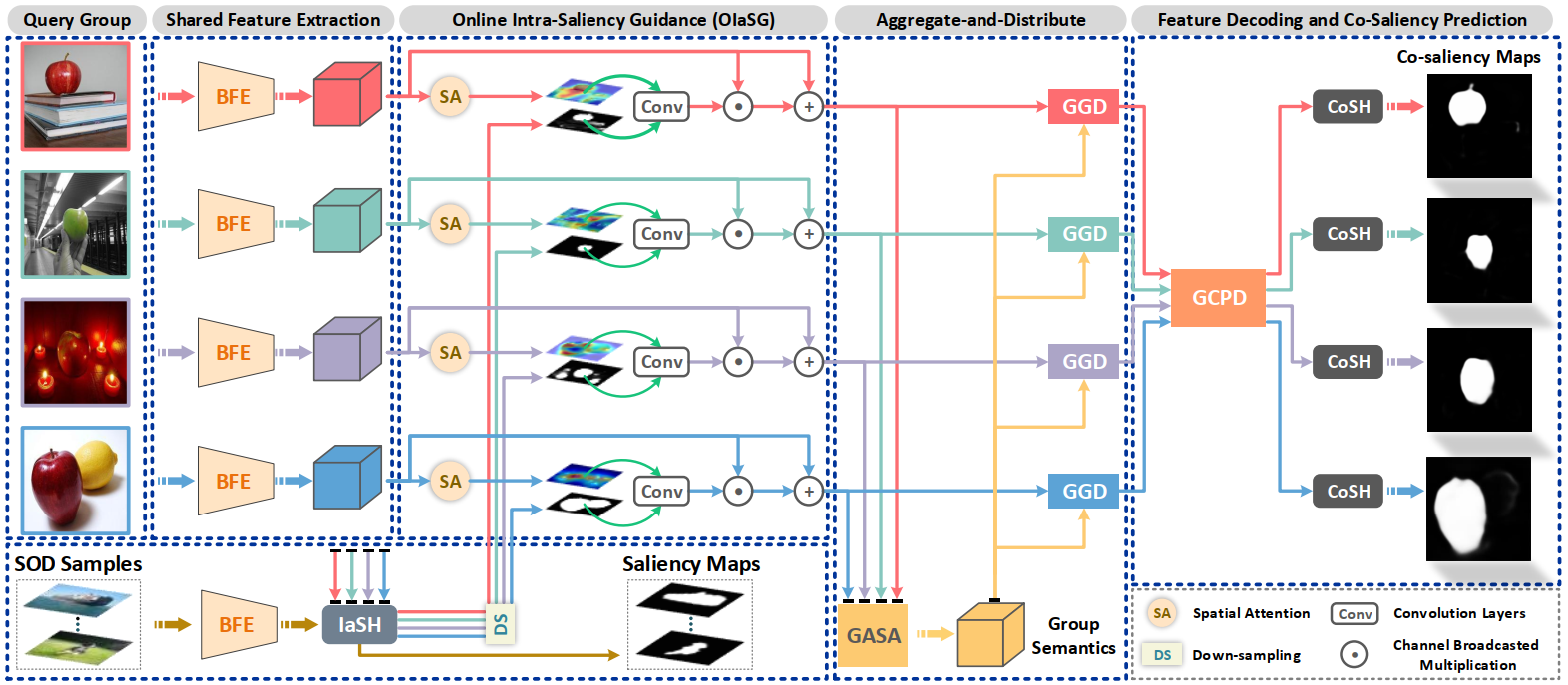
The flowchart of the proposed CoADNet. Given a query image group, we first obtain the deep features with a shared backbone feature extractor (BFE) and integrate learnable saliency priors with an OIaSG structure. The generated intra-saliency features are aggregated into group semantics through the group-attentional semantic aggregation (GASA) module, then they are further adaptively distributed to different individuals via the gated group distribution (GGD) module to learn co-saliency features. In the end, a group consistency-preserving decoder (GCPD) followed by a co-saliency head (CoSH) is used to predict consistent and full-resolution co-saliency maps.
Highlights
The proposed CoADNet provides some insights and improvements in terms of modeling and exploiting inter-image relationships in the CoSOD workflow, and produces more accurate and consistent co-saliency results on four prevailing co-saliency benchmark datasets.
We design an online intra-saliency guidance (OIaSG) module for supplying saliency prior knowledge, which is jointly optimized to generate trainable saliency guidance information. In this way, the network dynamically learns how to combine the saliency cues with deep individual features, which has better flexibility and expansibility in providing reliable saliency priors.
We propose a two-stage aggregate-and-distribute architecture to learn group-wise correspondences and co-saliency features. In the first stage, a group-attentional semantic aggregation (GASA) module is proposed to model inter-image relationships with long-range semantic dependencies. In the second stage, we propose a gated group distribution (GGD) module to distribute the learned group semantics to different individuals in a dynamic and unique way.
A group consistency preserving decoder (GCPD) is designed to replace conventional up-sampling or deconvolution driven feature decoding structures, which exploits more sufficient inter-image constraints to generate full-resolution co-saliency maps while maintaining group-wise consistency.
Qualitative Evaluation
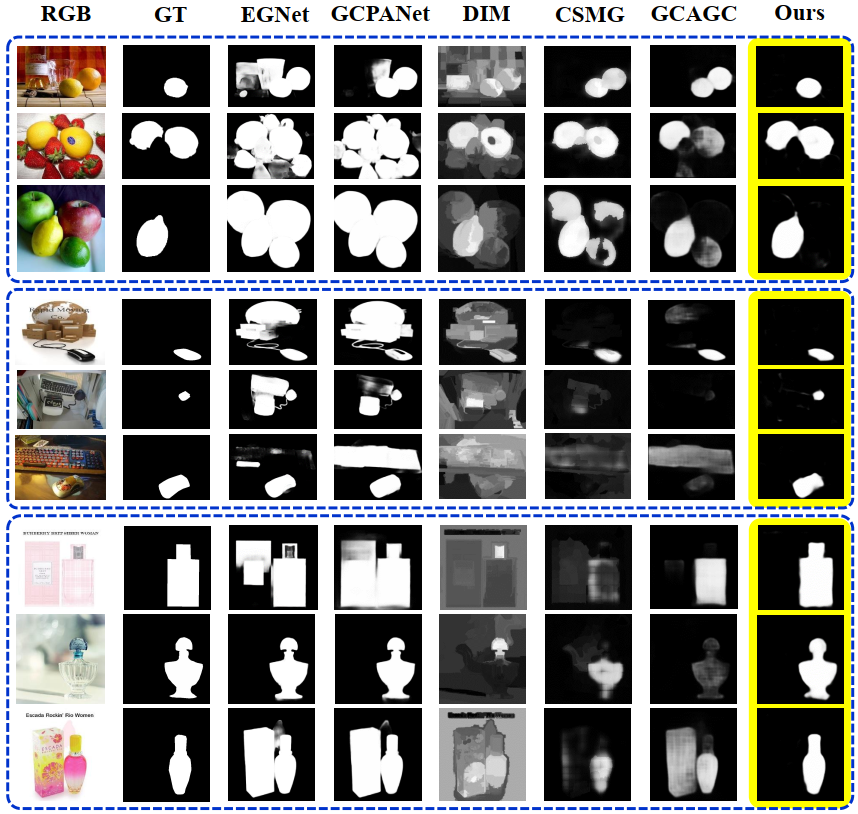
Quantitative Evaluation
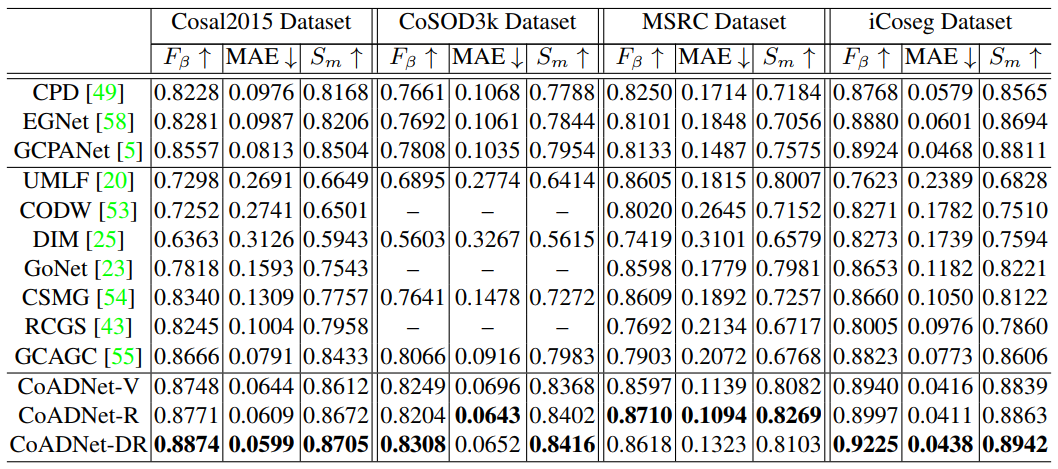
Quantitative comparisons over four benchmark datasets. The bold numbers indicate the best performance on the corresponding dataset. '-V', '-R' and '-DR' mean the VGG16, ResNet-50, and Dilated ResNet-50 backbones, respectively.
Citation
@inproceedings{CoADNet, title={{CoADNet}: Collaborative aggregation-and-distribution networks for co-salient object detection}, author={Zhang, Qijian and Cong, Runmin and Hou, Junhui and Li, Chongyi and Zhao, Yao}, booktitle={Proc. NeurIPS}, pages={6959-6970}, year={2020} }
Contact
If you have any questions, please contact Runmin Cong at rmcong@bjtu.edu.cn.