Dense Attention Fluid Network for Salient
Object Detection in Optical Remote Sensing Images
2 Beijing Jiaotong University, Beijing, China
3 Nanyang Technological University, Singapore
4 Nankai University, Tianjin, China
5 Jiangxi University of Finance and Economics, Nanchang, China
6 Institute of Information Engineering, Chinese Academy of Sciences, Beijing, China
Abstract
Despite the remarkable advances in visual saliency analysis for natural scene images (NSIs), salient object detection (SOD) for optical remote sensing images (RSIs) still remains an open and challenging problem. In this paper, we propose an end-to-end Dense Attention Fluid Network (DAFNet) for SOD in optical RSIs. A Global Context-aware Attention (GCA) module is proposed to adaptively capture long-range semantic context relationships, and is further embedded in a Dense Attention Fluid (DAF) structure that enables shallow attention cues flow into deep layers to guide the generation of high-level feature attention maps. Specifically, the GCA module is composed of two key components, where the global feature aggregation module achieves mutual reinforcement of salient feature embeddings from any two spatial locations, and the cascaded pyramid attention module tackles the scale variation issue by building up a cascaded pyramid framework to progressively refine the attention map in a coarse-to-fine manner. In addition, we construct a new and challenging optical RSI dataset for SOD that contains 2,000 images with pixel-wise saliency annotations, which is currently the largest publicly available benchmark. Extensive experiments demonstrate that our proposed DAFNet significantly outperforms the existing state-of-the-art SOD competitors.
Pipeline
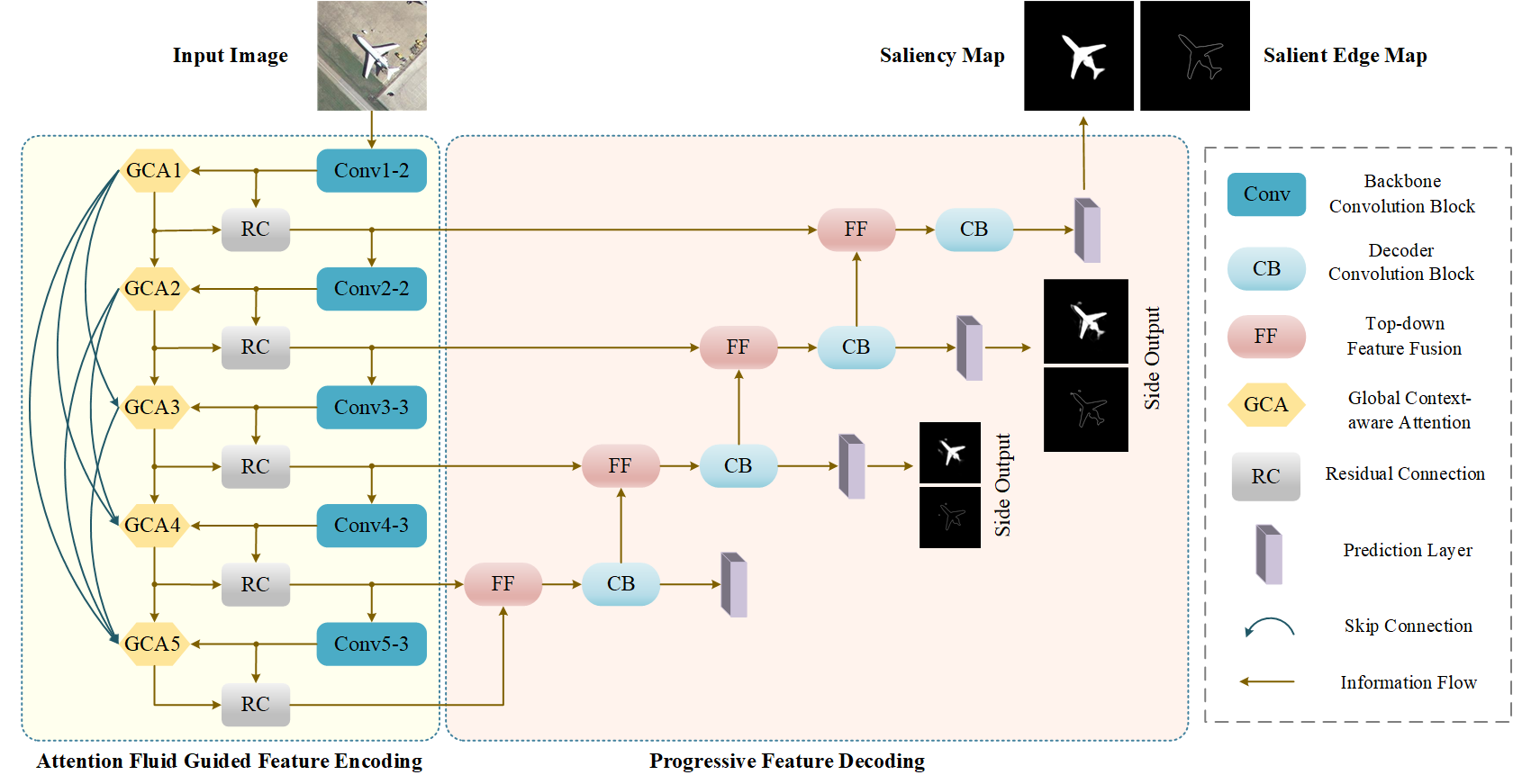
The overall architecture of our proposed DAFNet, including the Attention Fluid Guided Feature Encoding and Progressive Feature Decoding. The feature encoder consists of: 1) an attention fluid where low-level attention maps flow into deeper layers to guide the generation of high-level attentions, and 2) a feature fluid that generates hierarchical feature representations with stronger discriminative ability by incorporating attention cues mined from the corresponding global context-aware attention modules. The feature decoder employs a progressive top-down feature fusion strategy to produce saliency predictions at different feature scales. Note that we do not use the top-most side outputs for deep supervision in that the feature resolution is very low and hinders the depiction of detailed object structures. The FF unit involves up-sampling the high-level feature map and performing channel alignment via 1X1 convolutions, and then adding it to the low-level feature map. The CB unit is designed as a bottleneck convolution block with non-linearities to further integrate fusion feature information. The prediction layer consumes the decoded features to produce the corresponding saliency map.
EORSSD Dataset
In literature [1], an optical remote sensing saliency detection (ORSSD) dataset with pixel-wise ground truth is built, including 600 training images and 200 testing images. This is the first publicly available dataset for the RSI SOD task,
which bridges the gap between theory and practice in SOD for optical RSIs, but the amount of data is still slightly insufficient to train a deep learning based model.
To enlarge the size and enrich the variety of the dataset, we extend our ORSSD dataset to a larger one named Extended ORSSD (EORSSD) dataset with 2,000 images and
the corresponding pixel-wise ground truth, which includes many semantically meaningful but challenging images. Based on the ORSSD dataset, we collect additional
1,200 optical remote sensing images from the free Google Earth software, covering more complicated scene types, more challenging object attributes, and more
comprehensive real-world circumstances. For clarity, the EORSSD dataset is divided into two parts, i.e., 1,400 images for training and 600 images for testing.
[1] Chongyi Li, Runmin Cong, Junhui Hou, Sanyi Zhang, Yue Qian, and Sam Kwong, Nested network with two-stream pyramid for salient object detection in optical
remote sensing images, IEEE Transactions on Geoscience and Remote Sensing, vol. 57, no. 11, pp. 9156-9166, 2019.
[----Project Page----]

Visualization of the more challenging EORSSD dataset. The first row shows the optical RSI, and the second row exhibits the corresponding ground truth. (a) Challenge in the number of salient objects. (b) Challenge in small salient objects. (c) Challenge in new scenarios. (d) Challenge in interferences from imaging. (e) Challenge in specific circumstances.
Highlights
An end-to-end Dense Attention Fluid Network (DAFNet) is proposed to achieve SOD in optical RSIs, equipped with a Dense Attention Fluid (DAF) structure that is decoupled from the backbone feature extractor and the Global Context-aware Attention (GCA) mechanism.
The DAF structure is designed to combine multi-level attention cues, where shallow-layer attention cues flow into the attention units of deeper layers so that low-level attention cues could be propagated as guidance information to enhance the high-level attention maps.
The GCA mechanism is proposed to model the global-context semantic relationships through a global feature aggregation module, and further tackle the scale variation problem under a cascaded pyramid attention framework.
A large-scale benchmark dataset, named EORSSD dataset, containing 2,000 image samples and the corresponding pixel-wise annotations is constructed for the SOD task in optical RSIs. The proposed DAFNet consistently outperforms 15 state-of-the-art competitors in the experiments.
Results
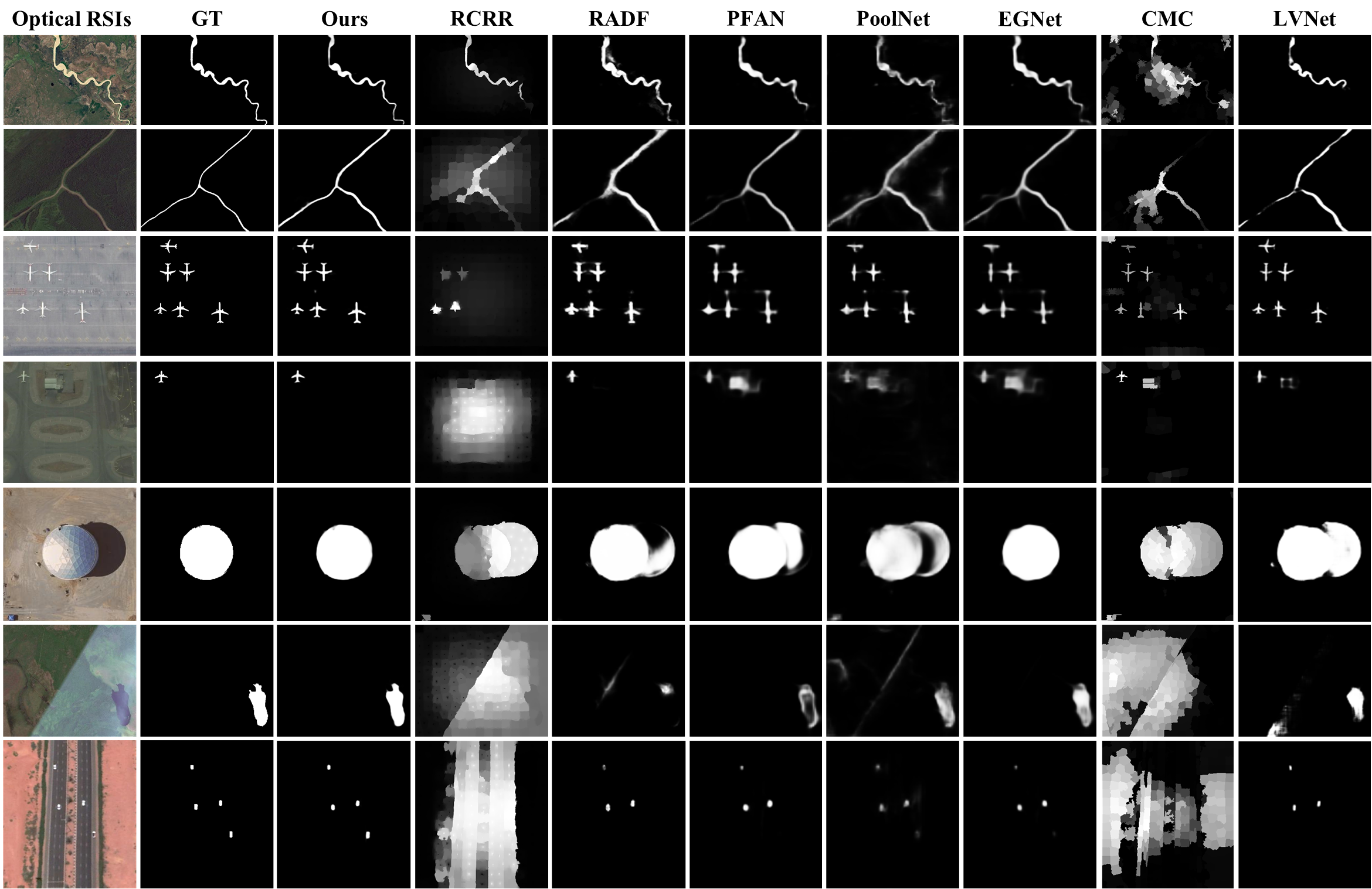
Citation
@article{DAFNet, title={Dense attention fluid network for salient object detection in optical remote sensing images}, author={Zhang, Qijian and Cong, Runmin and Li, Chongyi and Cheng, Ming-Ming and Fang, Yuming and Cao, Xiaochun and Zhao, Yao and Kwong, Sam}, journal={IEEE Transactions on Image Processing}, volume={30}, pages={1305-1317}, year={2021}, publisher={IEEE} }
Contact
If you have any questions, please contact Runmin Cong at rmcong@bjtu.edu.cn.