DPANet: Depth Potentiality-Aware Gated Attention Network for RGB-D Salient Object Detection
2 Beijing Jiaotong University, Beijing, China
3 Institute of Computing Technology, Chinese Academy of Sciences, Beijing, China
Abstract
There are two main issues in RGB-D salient object detection: (1) how to effectively integrate the complementarity from the cross-modal RGB-D data; (2) how to prevent the contamination effect from the unreliable depth map. In fact, these two problems are linked and intertwined, but the previous methods tend to focus only on the first problem and ignore the consideration of depth map quality, which may yield the model fall into the sub-optimal state. In this paper, we address these two issues in a holistic model synergistically, and propose a novel network named DPANet to explicitly model the potentiality of the depth map and effectively integrate the cross-modal complementarity. By introducing the depth potentiality perception, the network can perceive the potentiality of depth information in a learning-based manner, and guide the fusion process of two modal data to prevent the contamination occurred. The gated multi-modality attention module in the fusion process exploits the attention mechanism with a gate controller to capture long-range dependencies from a cross-modal perspective. Experimental results compared with 16 state-of-the-art methods on 8 datasets demonstrate the validity of the proposed approach both quantitatively and qualitatively.
Pipeline
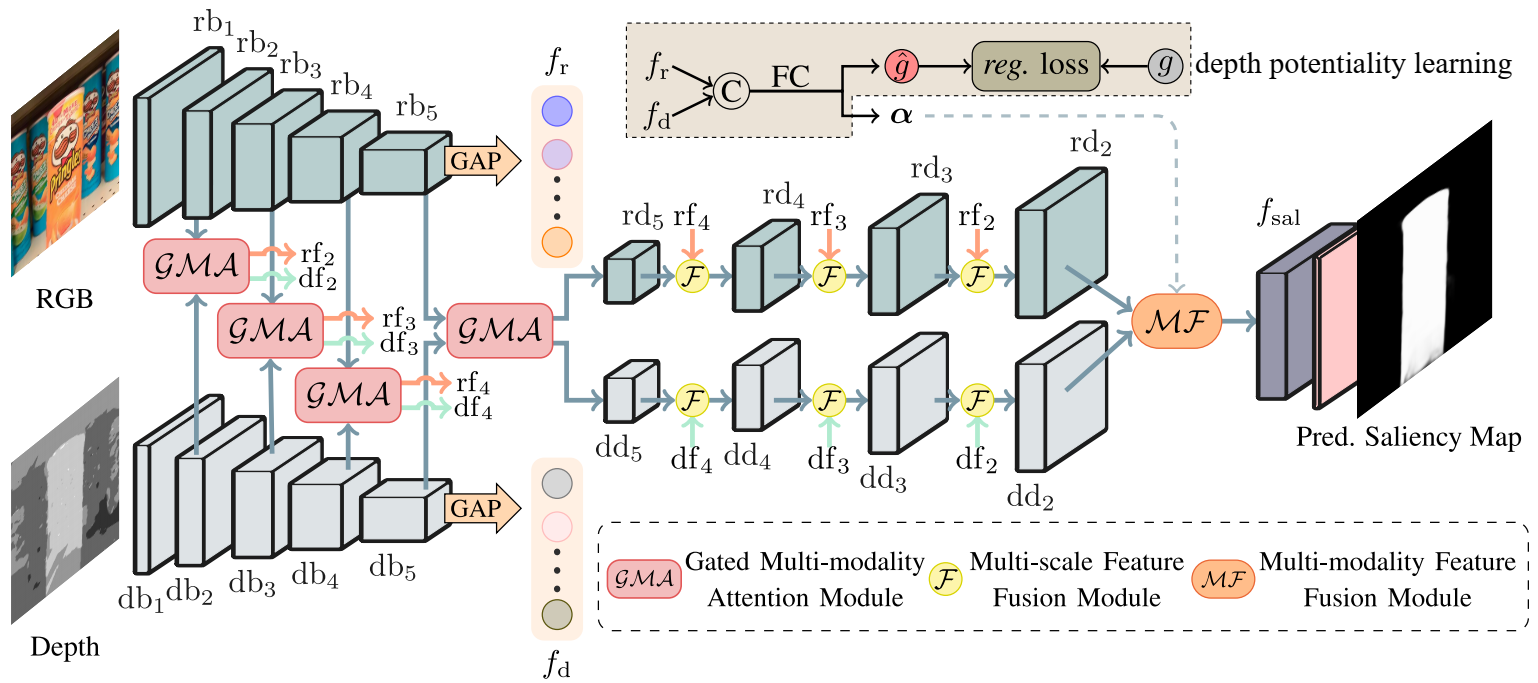
Architecture of DPANet. For better visualization, we only display the modules and features of each stage. rbi, dbi (i = 1, 2, ..., 5) denote the features generated by the backbone of the two branches respectively, and rdi, ddi (i = 5, 4, 3, 2) represent the features of decoder stage. rfi; dfi (i = 2, 3, 4, 5, rf5 = rd5, df5 = dd5) refer to the output of the GMA module. fsal is the generated final saliency map. 'C' and 'FC' refer to the concatenation and fully-connected layers respectively, and 'GAP' represents the global average pooling.
Highlights
For the first time, we address the unreliable depth map in the RGB-D SOD network in an end-to-end formulation, and propose the DPANet by incorporating the depth potentiality perception into the cross-modality integration pipeline.
Without increasing the training label (i.e., depth quality label), we model a task-orientated depth potentiality perception module that can adaptively perceive the potentiality of the input depth map, and further weaken the contamination from unreliable depth information.
We propose a GMA module to effectively aggregate the cross-modal complementarity of the RGB and depth images, where the spatial attention mechanism aims at reducing the information redundancy, and the gate function controller focuses on regulating the fusion rate of the cross-modal information.
Without any pre-processing (e.g., HHA) or post-processing (e.g., CRF) techniques, the proposed network outperforms 16 state-of-the-art methods on 8 RGB-D SOD datasets in quantitative and qualitative evaluations.
Qualitative Evaluation
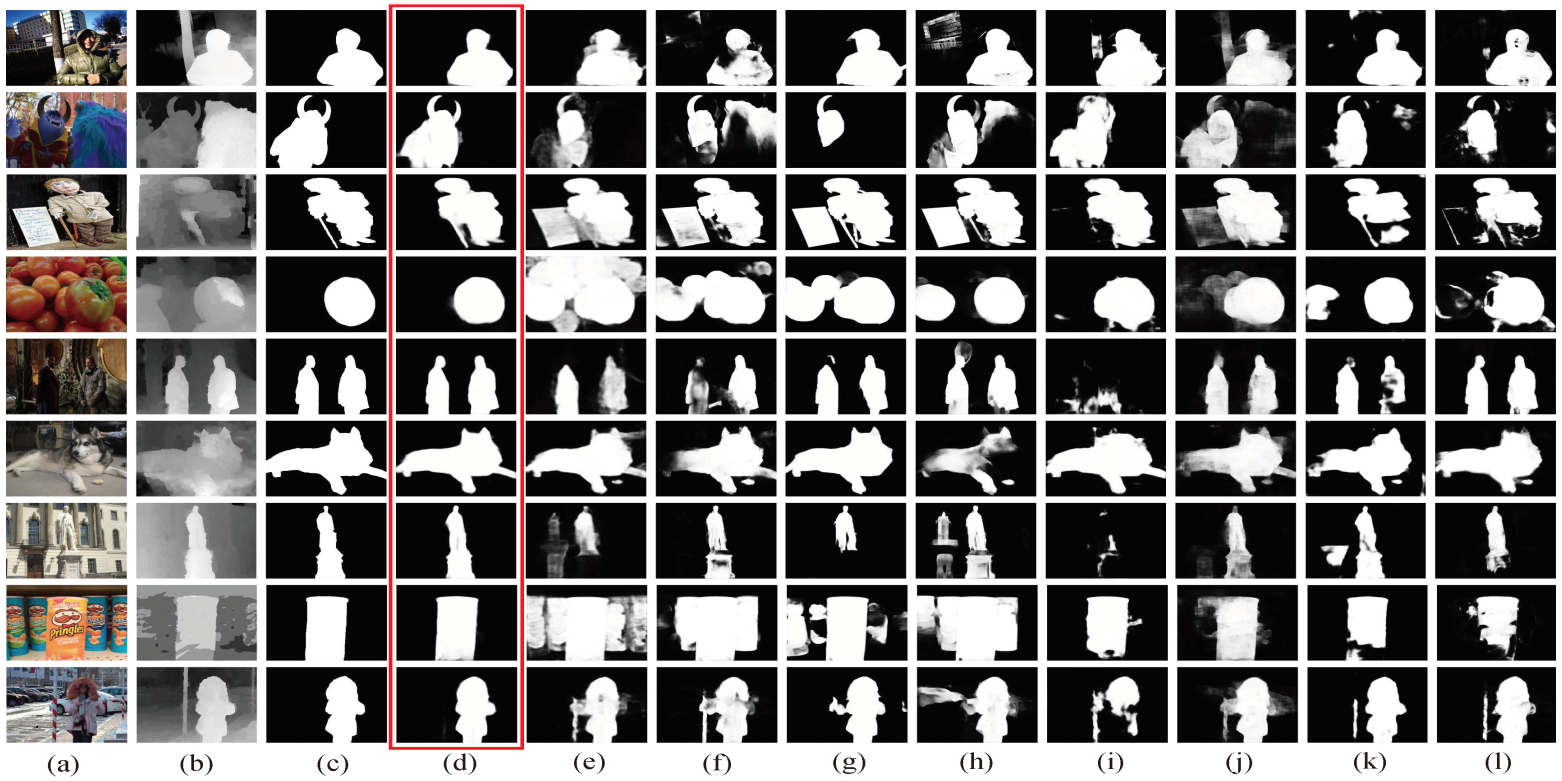
Qualitative comparison of the proposed approach with some state-of-the-art RGB and RGB-D SOD methods, in which our results are highlighted by a red box. (a) RGB image. (b) Depth map. (c) GT. (d) DPANet. (e) PiCAR. (f) PoolNet. (g) BASNet. (h) EGNet. (i) CPFP. (j) PDNet. (k) DMRA. (l) AF-Net.
Quantitative Evaluation
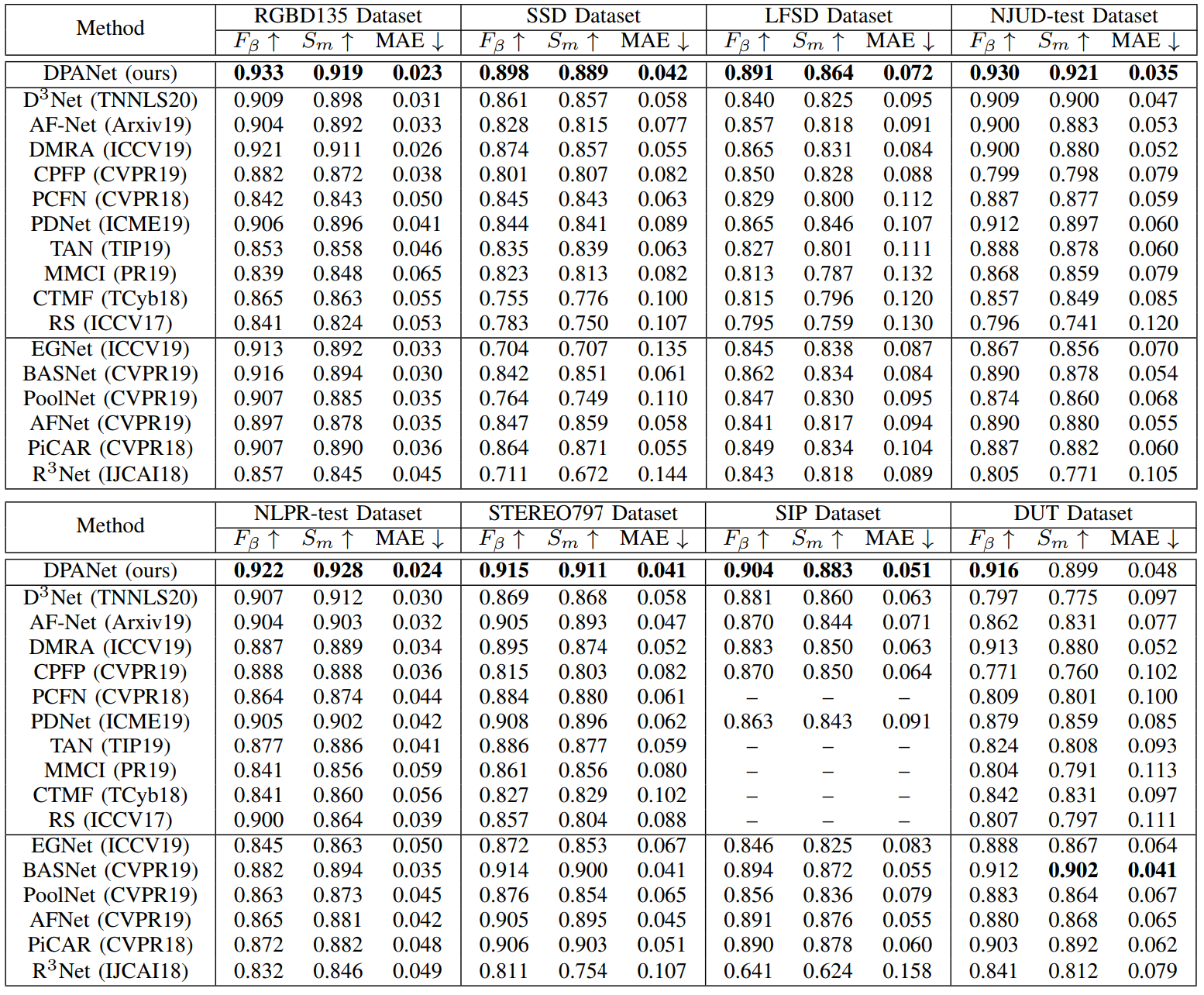
Citation
@article{DPANet, title={{DPANet}: Depth potentiality-aware gated attention network for {RGB-D} salient object detection}, author={Chen, Zuyao and Cong, Runmin and Xu, Qianqian and Huang, Qingming}, journal={IEEE Transactions on Image Processing}, year={2021}, publisher={IEEE} }
Contact
If you have any questions, please contact Runmin Cong at rmcong@bjtu.edu.cn.