A Weakly Supervised Learning Framework for Salient Object Detection via Hybrid Labels
2 Ningbo University, Ningbo, China
3 City University of Hong Kong, China
Abstract
Fully-supervised salient object detection (SOD) methods have made great progress, but such methods often rely on a large number of pixel-level annotations, which are time-consuming and labour-intensive. In this paper, we focus on a new weakly-supervised SOD task under hybrid labels, where the supervision labels include a large number of coarse labels generated by the traditional unsupervised method and a small number of real labels. To address the issues of label noise and quantity imbalance in this task, we design a new pipeline framework with three sophisticated training strategies. In terms of model framework, we decouple the task into label refinement sub-task and salient object detection sub-task, which cooperate with each other and train alternately. Specifically, the R-Net is designed as a two-stream encoder-decoder model equipped with Blender with Guidance and Aggregation Mechanisms (BGA), aiming to rectify the coarse labels for more reliable pseudo-labels, while the S-Net is a replaceable SOD network supervised by the pseudo labels generated by the current R-Net. Note that, we only need to use the trained S-Net for testing. Moreover, in order to guarantee the effectiveness and efficiency of network training, we design three training strategies, including alternate iteration mechanism, group-wise incremental mechanism, and credibility verification mechanism. Experiments on five SOD benchmarks show that our method achieves competitive performance against weakly-supervised/unsupervised methods both qualitatively and quantitatively.
Pipeline
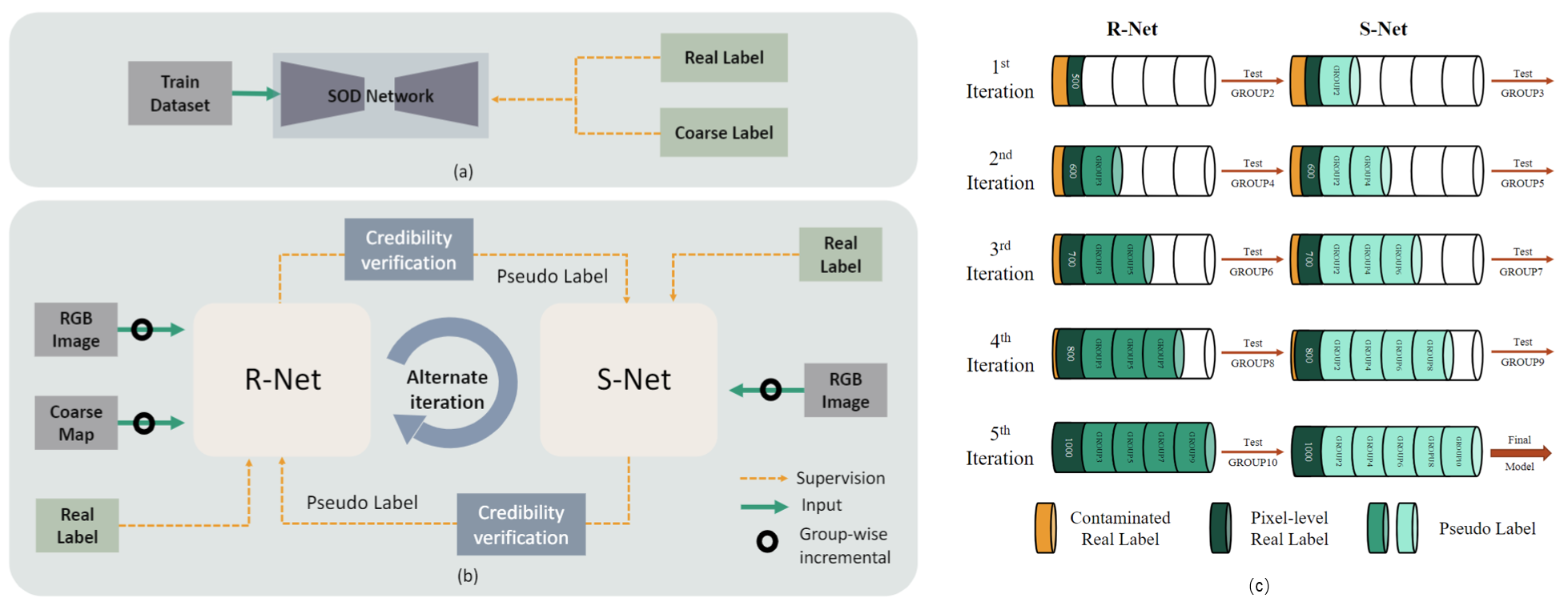
(a) A simple solution for training the SOD model with coarse and real labels. (b) The proposed alternate learning framework for weakly-supervised SOD task under the hybrid label, consisting of a Refine Network (R-Net) and a Saliency Network (S-Net). These two networks cooperate with each other and train alternately. During training, both networks employ a group-wise incremental mechanism to address the imbalance between real-labeled data and pseudo-labeled data, and use a credibility verification mechanism to ensure that the two networks can provide credible labels. (c) Training strategy for group update based on real labels and pseudo labels.
Model Details
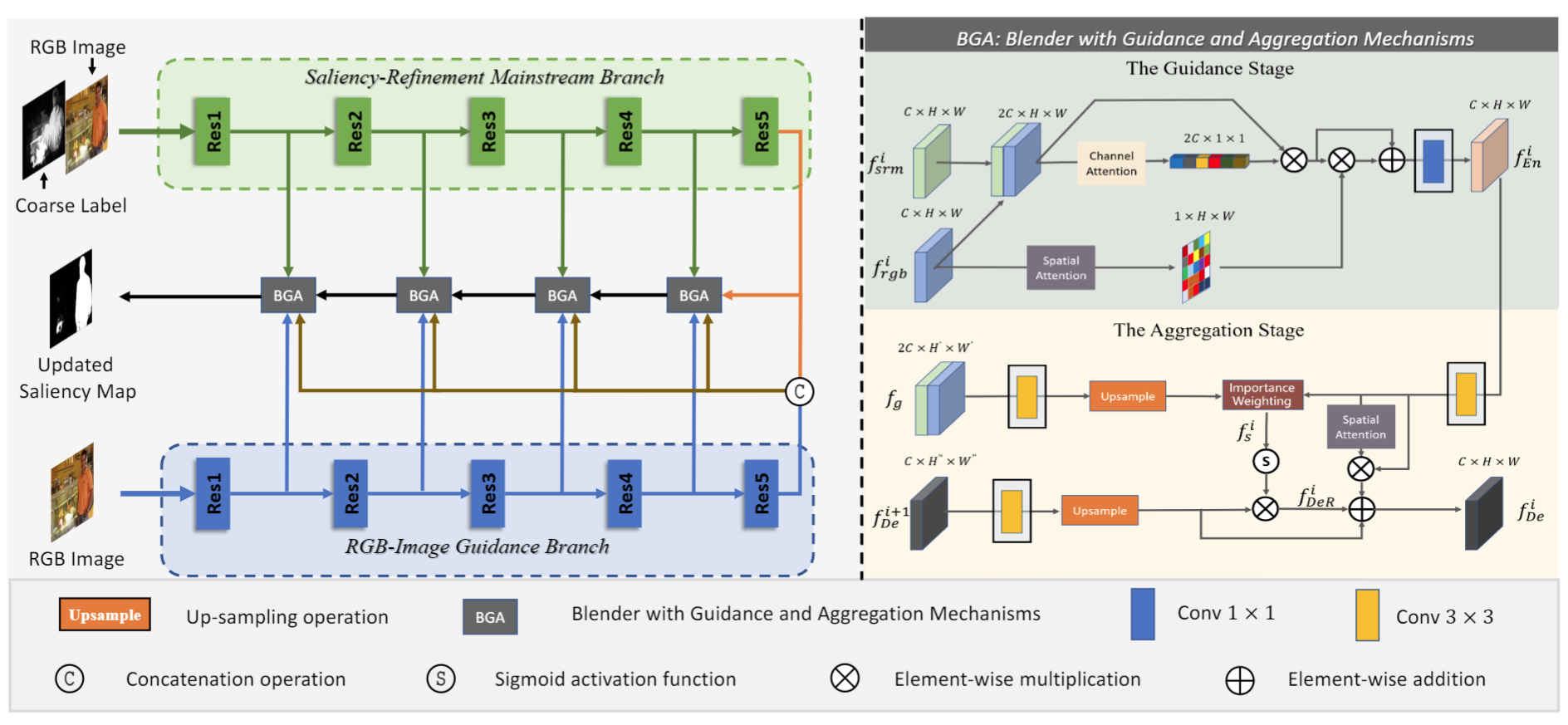
The overall framework of the proposed Refine Network (R-Net).
Highlights
For the first time, we launch a new weakly-supervised SOD task based on hybrid labels, with a large number of coarse labels and a small number of real labels as supervision. To this end, we decouple this task into two sub-tasks of coarse label refinement and salient object detection, and design the corresponding R-Net and S-Net. Moreover, our method achieves competitive performance on five widely used benchmark datasets using only one-tenth of the real labels in fully-supervised setting.
We design a BGA in the R-Net to achieve two-stage feature decoding, where the guidance stage is used to introduce the guidance information from the RGB-image guidance branch to guarantee a relatively robust performance baseline, and the aggregation stage is to dynamically integrate different levels of features according to their modification or supplementation roles.
In order to guarantee the effectiveness and efficiency of network training, from the perspective of quantity allocation, training method and reliability judgment, we design the alternate iteration mechanism, group-wise incremental mechanism, and credibility verification mechanism.
Qualitative Evaluation
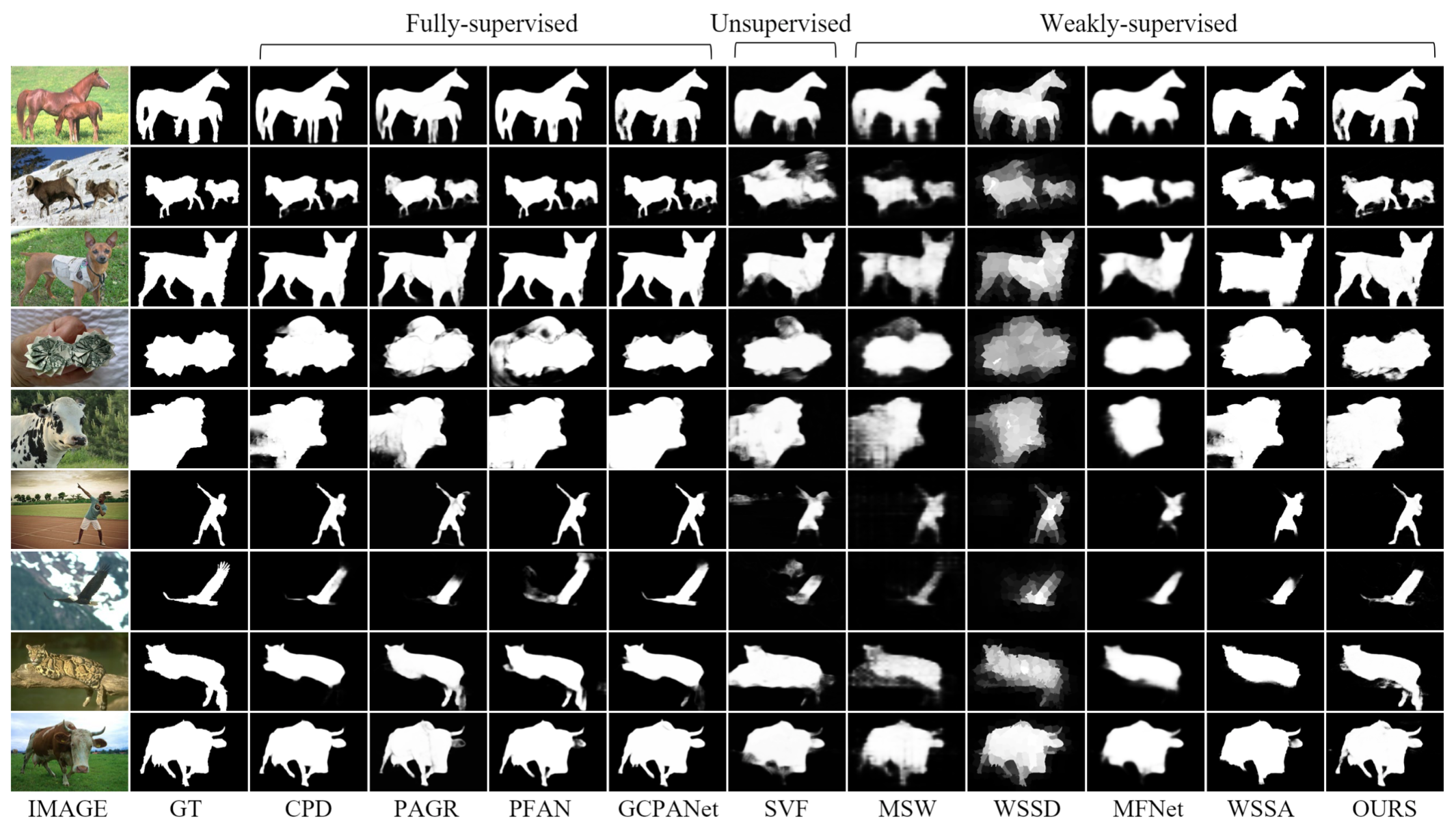
Visual comparisons with other state-of-the-art methods in various representative scenes.
Quantitative Evaluation
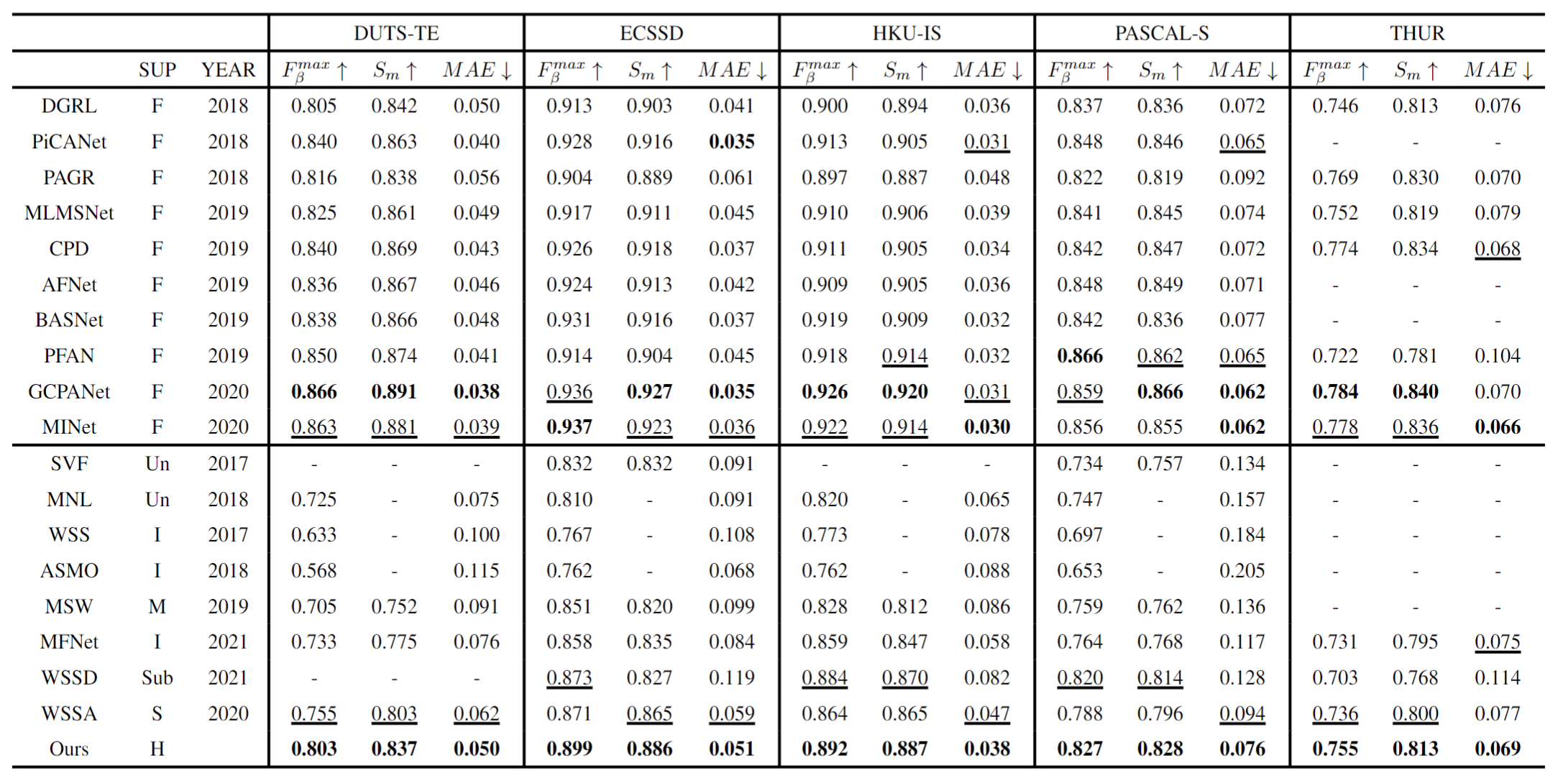
Quantitative results of different methods on five SOD benchmark datasets, ↑ and ↓ respectively indicate that the larger and smaller the score, the better. ‘F’ means fully supervision, ‘I’ means image-level weakly supervision, and ‘S’ means scribble-level weakly supervision, 'Sub' means subitizing supervision, ‘M’ means multi-source weakly supervision, ‘Un’ is for unsupervision, and ‘H’ denotes hybird supervision. The best performance is marked in BOLD, and the second best performance is marked in UNDERLINE.
Citation
@article{HybridSOD, title={A weakly supervised learning framework for salient object detection via hybrid labels}, author={Cong, Runmin and Qin, Qi and Zhang, Chen and Jiang, Qiuping and Wang, Shiqi and Zhao, Yao and Kwong, Sam }, journal={IEEE Transactions on Circuits and Systems for Video Technology}, year={2022}, publisher={IEEE} }
Contact
If you have any questions, please contact Runmin Cong at rmcong@bjtu.edu.cn.