Multi-Projection Fusion and Refinement Network for Salient Object Detection in 360° Omnidirectional Image
2 Tianjin University, Tianjin, China
3 University of Chinese Academy of Sciences, Beijing, China
4 City University of Hong Kong, China
Abstract
Salient object detection (SOD) aims to determine the most visually attractive objects in an image. With the development of virtual reality technology, 360° omnidirectional image has been widely used, but the SOD task in 360° omnidirectional image is seldom studied due to its severe distortions and complex scenes. In this paper, we propose a Multi-Projection Fusion and Refinement Network (MPFR-Net) to detect the salient objects in 360° omnidirectional image. Different from the existing methods, the equirectangular projection image and four corresponding cube-unfolding images are embedded into the network simultaneously as inputs, where the cube-unfolding images not only provide supplementary information for equirectangular projection image, but also ensure the object integrity of the cube-map projection. In order to make full use of these two projection modes, a Dynamic Weighting Fusion (DWF) module is designed to adaptively integrate the features of different projections in a complementary and dynamic manner from the perspective of inter and intra features. Furthermore, in order to fully explore the way of interaction between encoder and decoder features, a Filtration and Refinement (FR) module is designed to suppress the redundant information between the feature itself and the feature. Experimental results on two omnidirectional datasets demonstrate that the proposed approach outperforms the state-of-the-art methods both qualitatively and quantitatively.
Pipeline
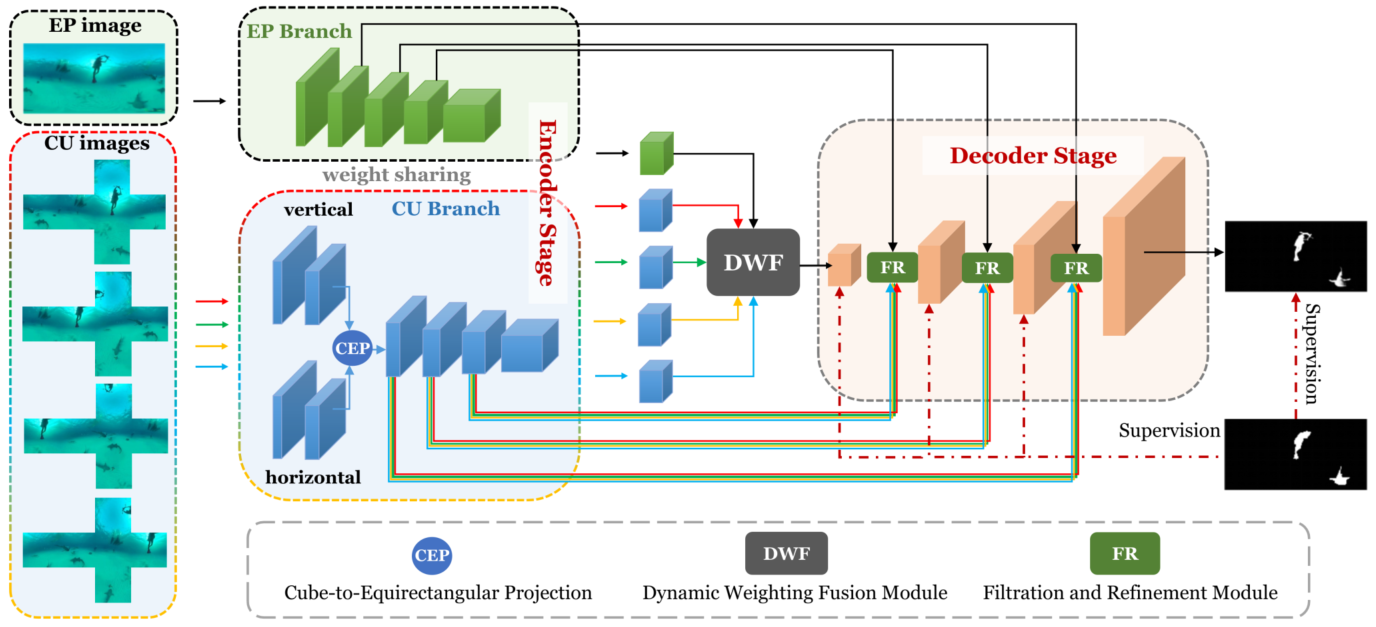
The overall pipeline of the proposed MPFR-Net. Equirectangular image and four cube-unfolding images are used as the inputs of the encoder-decoder network. The encoder is a weight-shared ResNet-50 composed of five convolutional blocks. In the feature decoding process, the equirectangular features and cube-unfolding features are firstly fused by the DWF module in a dynamic weighing manner. Then, in each level of decoding, the redundant information in features is filtered and refined by the FR module.
Highlights
A Multi-Projection Fusion and Refinement Network (MPFR-Net) is proposed to achieve salient object detection in 360° omnidirectional images, which introduces four cube-unfolding images through cube-map projection to supplement the corresponding equirectangular image.
A Dynamic Weighting Fusion (DWF) module is designed to adaptively integrate the multi-projection features from the perspective of inter and intra features in a complementary and dynamic manner.
A Filtration and Refinement (FR) module is presented to update the encoder and decoder features through the filtration scheme, and to suppress the redundant information in the fusion process through the refinement scheme.
Qualitative Evaluation
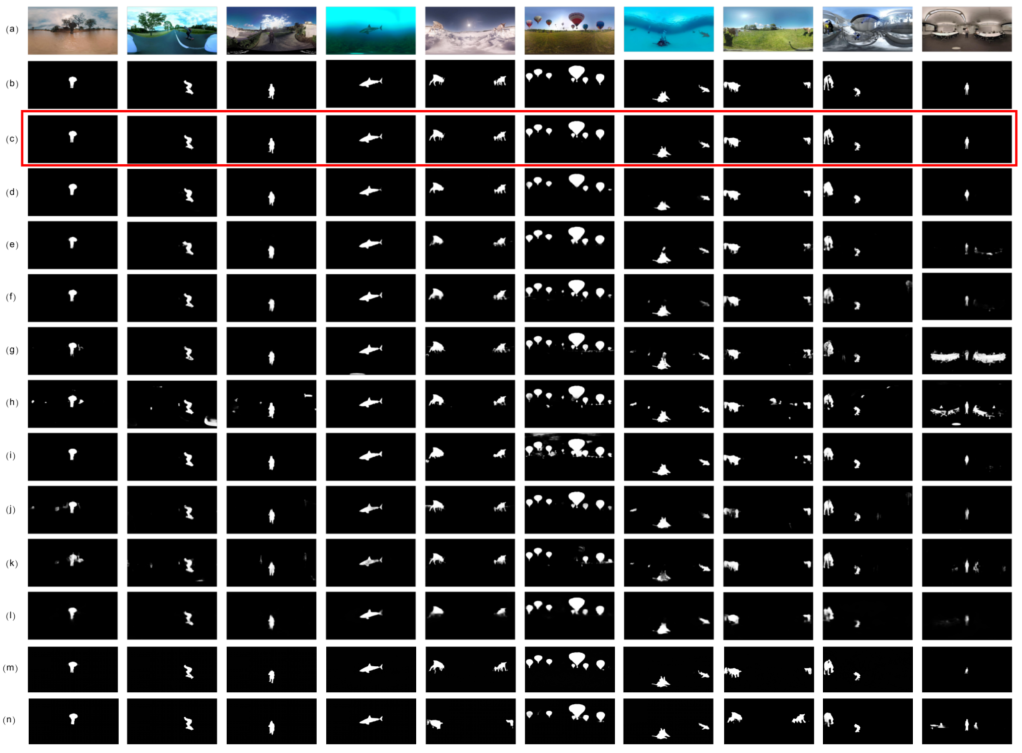
Visual comparisons with other state-of-the-art methods in various representative scenes. (a) Equirectangular images. (b) Ground truth. (c) Result of our proposed method (marked in red box). (d)-(n) Saliency maps generated by FANet, DDS, GCPANet, ITSD, F3Net, MINet, HVPNet, SAMNet, CPDNet, BPFINet, CTDNet.
Quantitative Evaluation
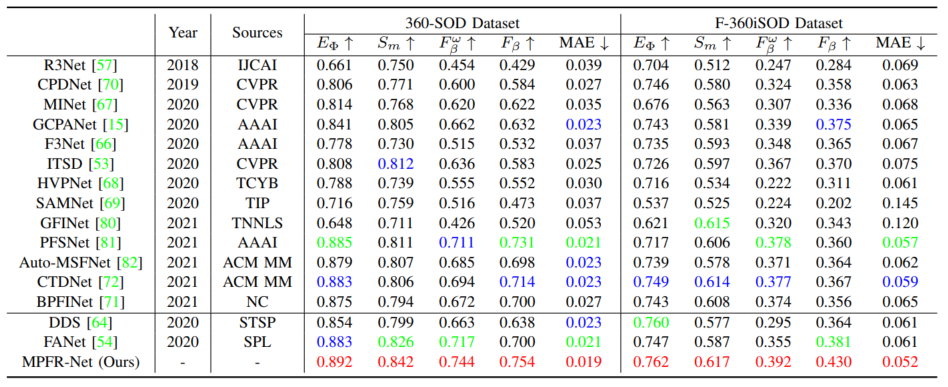
Quantitative comparison on two public datasets. From top to bottom: SOD methods on 2D images, SOD methods on 360° omnidirectional images and our method. ↑ and ↓ denote larger and smaller is better. The top three results are marked in red, green and blue.
Citation
@article{360SOD, title={Multi-projection fusion and refinement network for salient object detection in 360$^{\circ}$ omnidirectional image}, author={Cong, Runmin and Huang, Ke and Lei, Jianjun and Zhao, Yao and Huang, Qingming and Kwong, Sam }, journal={IEEE Transactions on Neural Networks and Learning Systems}, year={2022}, publisher={IEEE} }
Contact
If you have any questions, please contact Runmin Cong at rmcong@bjtu.edu.cn.