PSNet: Parallel Symmetric Network for Video
Salient Object Detection
2 Tianjin University, Tianjin, China
3 Shenzhen University, Beijing, China
4 City University of Hong Kong, China
Abstract
For the video salient object detection (VSOD) task, how to excavate the information from the appearance modality and the motion modality has always been a topic of great concern. The two-stream structure, including an RGB appearance stream and an optical flow motion stream, has been widely used as a typical pipeline for VSOD tasks, but the existing methods usually only use motion features to unidirectionally guide appearance features or adaptively but blindly fuse two modality features. However, these methods underperform in diverse scenarios due to the uncomprehensive and unspecific learning schemes. In this paper, following a more secure modeling philosophy, we deeply investigate the importance of appearance modality and motion modality in a more comprehensive way and propose a VSOD network with up and down parallel symmetry, named PSNet. Two parallel branches with different dominant modalities are set to achieve complete video saliency decoding with the cooperation of the Gather Diffusion Reinforcement (GDR) module and Cross-modality Refinement and Complement (CRC) module. Finally, we use the Importance Perception Fusion (IPF) module to fuse the features from two parallel branches according to their different importance in different scenarios. Experiments on four dataset benchmarks demonstrate that our method achieves desirable and competitive performance.
Pipeline
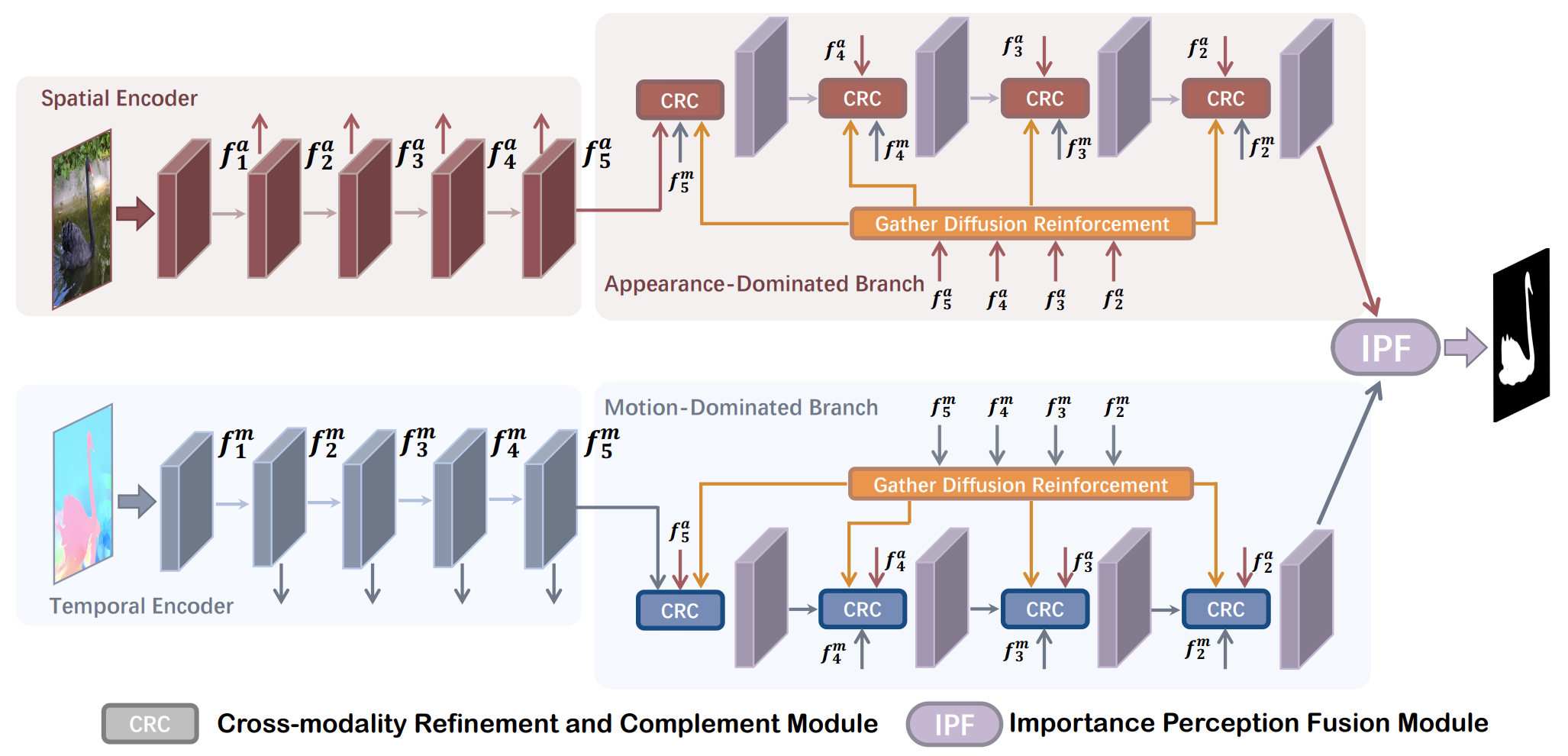
The flowchart of the proposed Parallel Symmetric Network (PSNet) for video salient object detection. We first extract the multi-level features from RGB images and optical flow maps via spatial encoder and temporal encoder respectively. Then, the appearance-dominated branch (top branch) and motion-dominated branch (bottom branch) are used to feature decoding. For each decoding, we use Gather Diffusion Reinforcement (GDR) module to perform cross-scale feature enhancement, and then use the Cross-modality Refinement and Complement (CRC) module to achieve cross-modality interaction with an explicit primary and secondary modality relationship. Finally, the Importance Perception Fusion (IPF) module is used to integrate the upper and lower branches by considering their different importance in different scenarios.
Highlights
Considering the adaptability of the network to different scenarios and the uncertainty of the role of different modalities, we propose a parallel symmetric network (PSNet) for VSOD that simultaneously models the importance of two modality features in an explicit way.
We propose a GDR module in each branch to perform multi-scale content enhancement for dominant features and design a CRC module to achieve cross-modality interaction, where the auxiliary features are applied to refine and supplement dominant features.
Experimental results on four mainstream datasets demonstrate that our PSNet outperforms 25 state-of-the-art methods both quantitatively and qualitatively.
Qualitative Evaluation
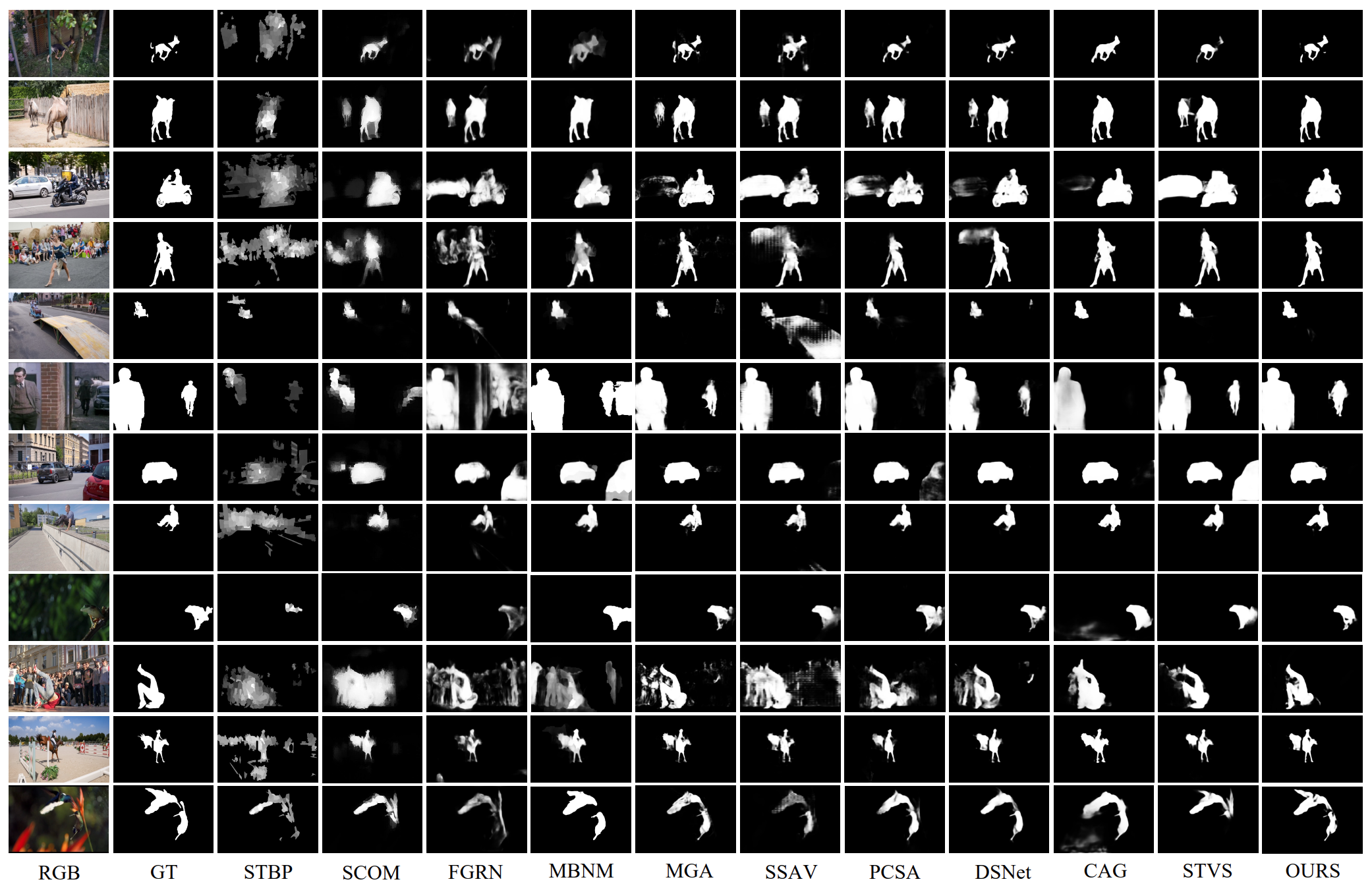
The visualization results of different video salient object detection methods.
Quantitative Evaluation
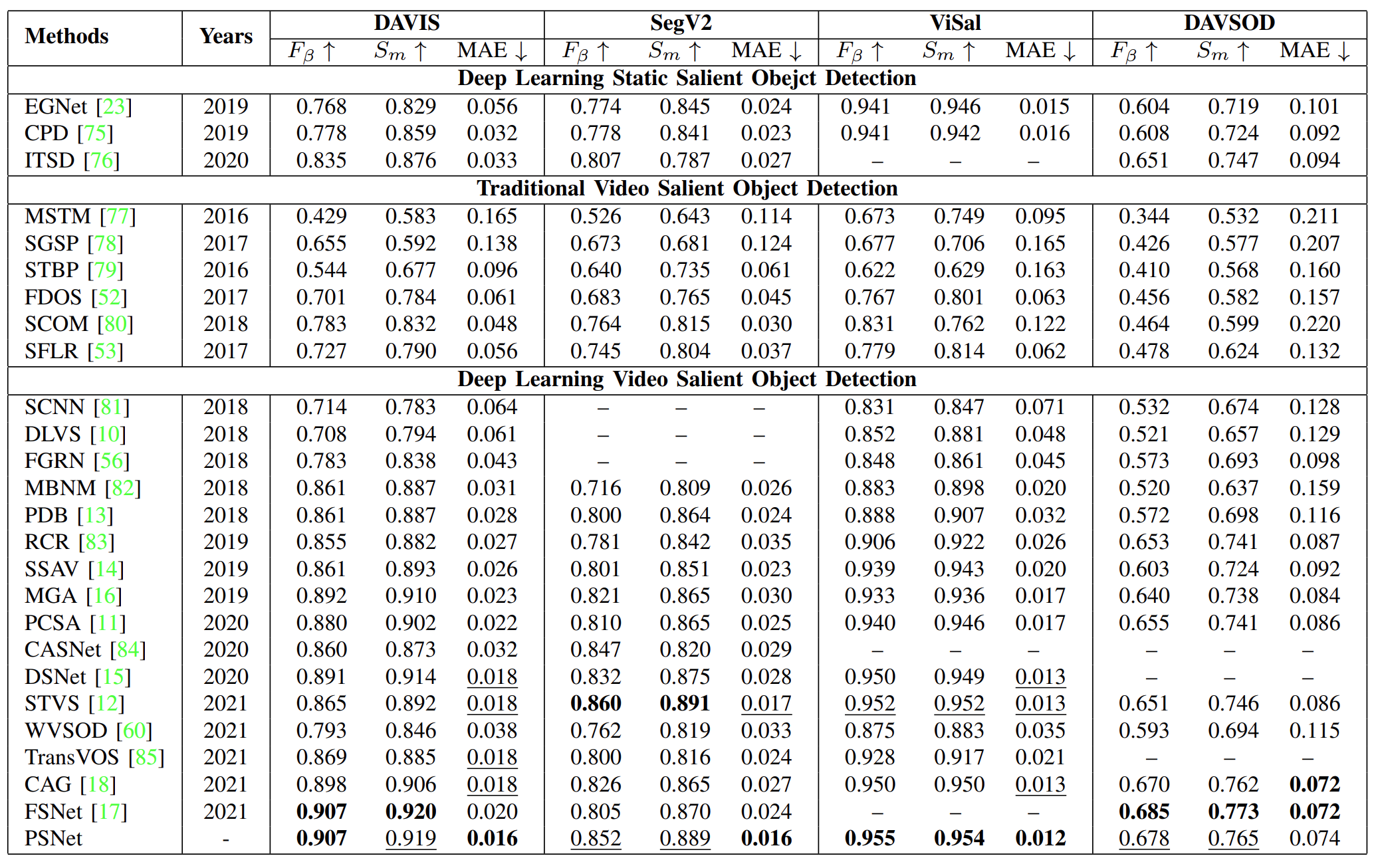
Quantitative results on the DAVIS, SegV2, DAVSOD, and ViSal datasets. The top two score was marked in bold and underline, respectively.
Citation
@article{PSNet, title={{PSNet}: Parallel Symmetric Network for Video Salient Object Detection}, author={Cong, Runmin and Song, Weiyu and Lei, Jianjun and Yue, Guanghui and Zhao, Yao and Kwong, Sam }, journal={IEEE Transactions on Emerging Topics in Computational Intelligence}, year={2022}, publisher={IEEE} }
Contact
If you have any questions, please contact Runmin Cong at rmcong@bjtu.edu.cn.