PUGAN: Physical Model-Guided Underwater Image Enhancement Using GAN with Dual-Discriminators
2 Beijing Jiaotong University, Beijing, China
3 Nankai University, Tianjin, China
4 University of Chinese Academy of Sciences, Beijing, China
5 City University of Hong Kong, China
Abstract
Due to the light absorption and scattering induced by the water medium, underwater images usually suffer from some degradation problems, such as low contrast, color distortion, and blurring details, which aggravate the difficulty of downstream underwater understanding tasks. Therefore, how to obtain clear and visually pleasant images has become a common concern of people, and the task of underwater image enhancement (UIE) has also emerged as the times require. Among existing UIE methods, Generative Adversarial Networks (GANs) based methods perform well in visual aesthetics, while the physical model-based methods have better scene adaptability. Inheriting the advantages of the above two types of models, we propose a physical model-guided GAN model for UIE in this paper, referred to as PUGAN. The entire network is under the GAN architecture. On the one hand, we design a Parameters Estimation subnetwork (Par-subnet) to learn the parameters for physical model inversion, and use the generated color enhancement image as auxiliary information for the Two-Stream Interaction Enhancement sub-network (TSIE-subnet). Meanwhile, we design a Degradation Quantization (DQ) module in TSIE-subnet to quantize scene degradation, thereby achieving reinforcing enhancement of key regions. On the other hand, we design the Dual-Discriminators for the style-content adversarial constraint, promoting the authenticity and visual aesthetics of the results. Extensive experiments on three benchmark datasets demonstrate that our PUGAN outperforms state-of-the-art methods in both qualitative and quantitative metrics.
Pipeline
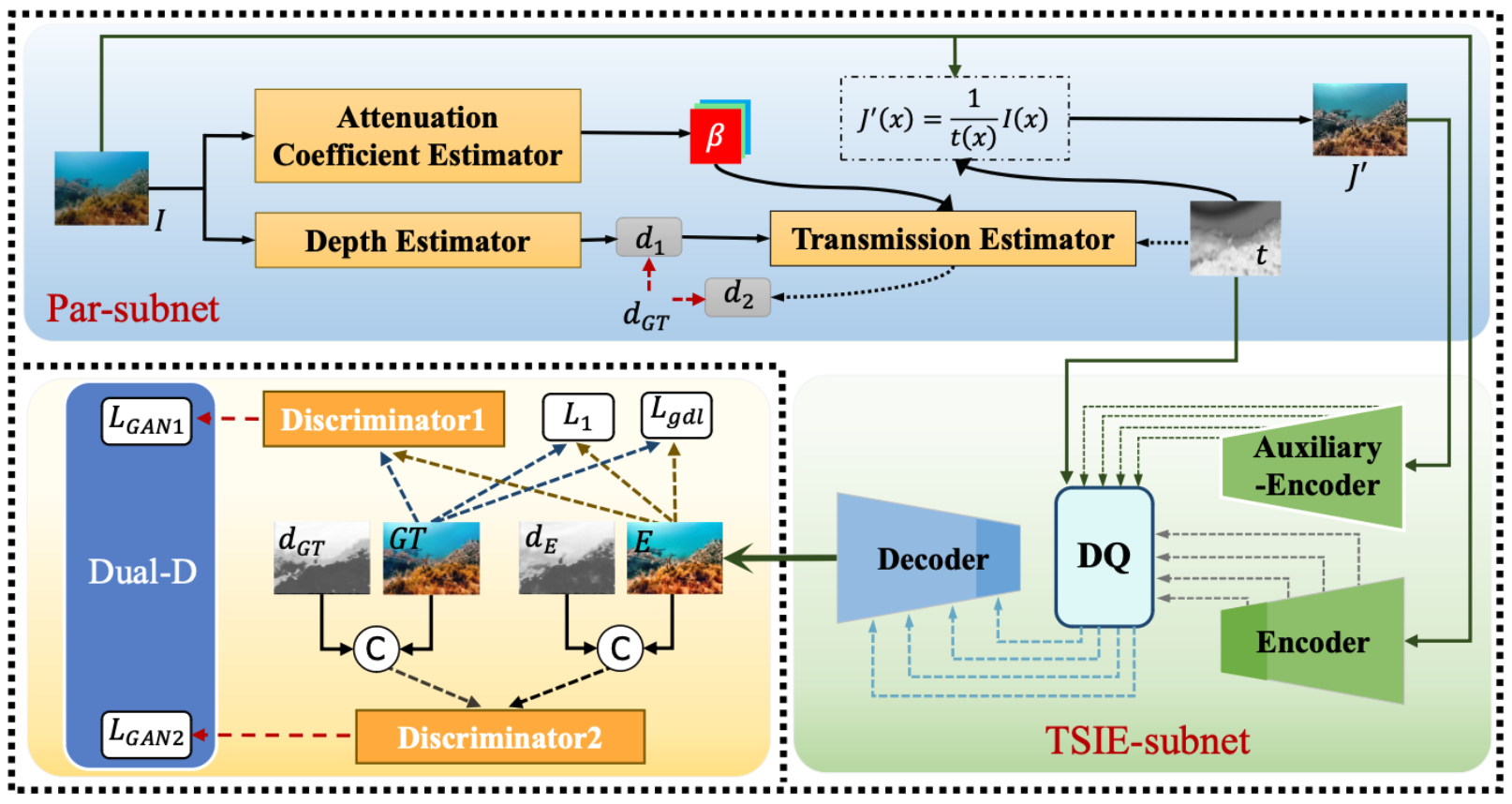
Overview of the proposed PUGAN for UIE task, including a Phy-G and a Dual-D under the GAN architecture. In the Phy-G, the Par-subnet is used to estimate the physical parameters (e.g., transmission map and attenuation coefficient) required for restoring a color-enhanced image J'. The TSIE-subnet aims to achieve the CNN-based end-to-end enhancement, where a degradation quantization (DQ) module is used to quantify the distortion degree of the scene, thereby guiding and generating the final enhanced underwater image E. The objective function consists of four parts, including global similarity loss, perceptual loss, style adversarial loss, and content adversarial loss.
Highlights
Considering the respective advantages of the physical model and the GAN model for the UIE task, we propose a Physical Model-Guided framework using GAN with Dual-Discriminators (PUGAN), consisting of a Phy-G and a Dual-D. Extensive experiments on three benchmark datasets demonstrate that our PUGAN outperforms state-of-the-art methods in both qualitative and quantitative metrics.
We design two subnetworks in the Phy-G, including the Par-subnet and the TSIE-subnet, for the parameter estimation of physical model and the physical model-guided CNN-based enhancement, respectively. On the one hand, we introduce an intermediate variable in the Par-subnet, i.e., depth, to enable effective estimation of the transmission map. On the other hand, we propose a DQ module in TSIE-subnet to quantify the distortion degrees and achieve targeted encoder feature reinforcing.
In addition to the pixel-level global similarly loss and perceptual loss, we design the style-content adversarial loss in the Dual-D to constrain the style and content of the enhanced underwater image to be realistic.
Qualitative Evaluation
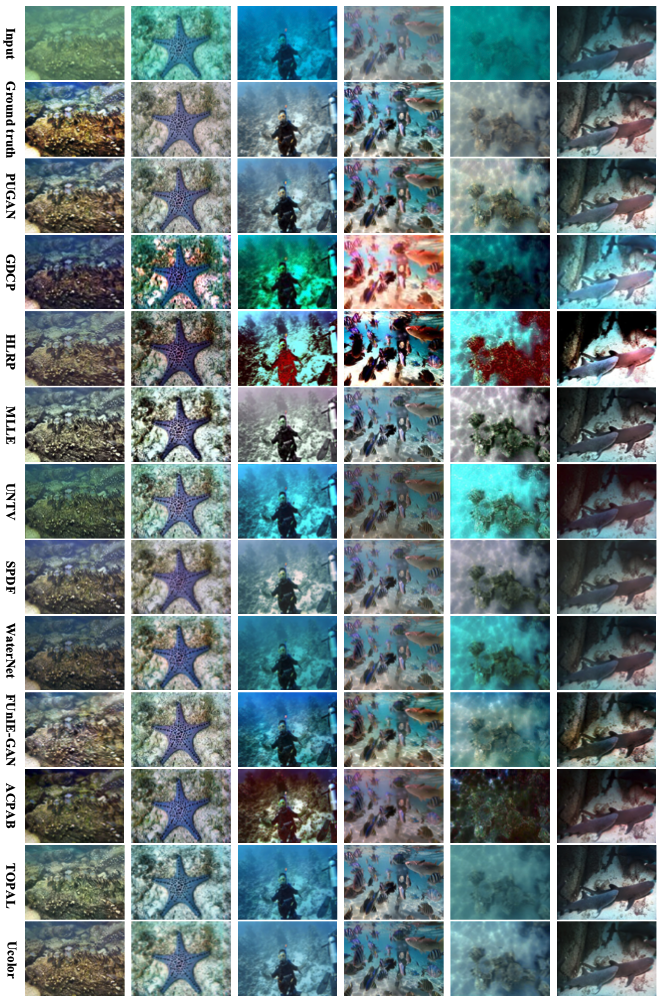
Quantitative Evaluation
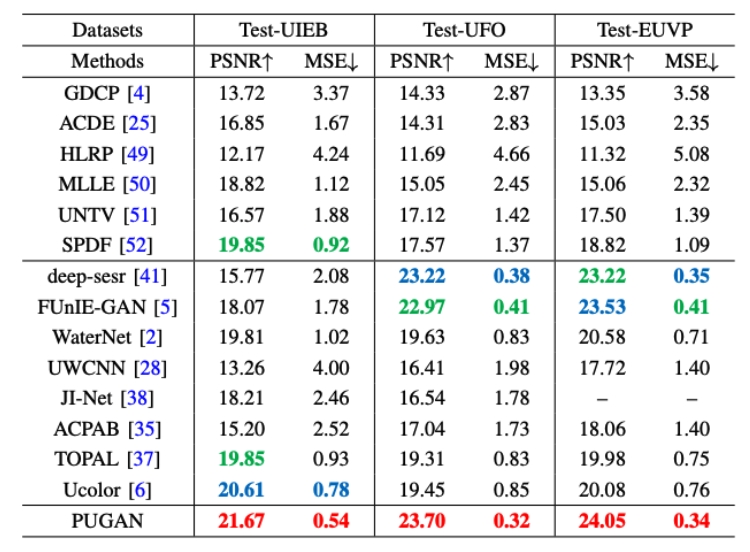
Citation
@inproceedings{PUGAN, author={Cong, Runmin and Yang, Wenyu and Zhang, Wei and Li, chongyi and Guo, Chun-Le and Huang, Qingming and Kwong, Sam }, title= {{PUGAN}: Physical model-guided underwater image enhancement using {GAN} with dual- discriminators}, journal={IEEE Transactions on Image Processing}, volume={32}, pages={4472-4485}, year={2023} }
Contact
If you have any questions, please contact Runmin Cong at rmcong@sdu.edu.cn.