RRNet: Relational Reasoning Network with Parallel Multi-scale Attention for Salient Object Detection in Optical Remote Sensing Images
2 Hunan University, Changsha, China
3 Sun Yat-sen University, Guangzhou, China
4 City University of Hong Kong, China
Abstract
Salient object detection (SOD) for optical remote sensing images (RSIs) aims at locating and extracting visually distinctive objects/regions from the optical RSIs. Despite some saliency models were proposed to solve the intrinsic problem of optical RSIs (such as complex background and scale-variant objects), the accuracy and completeness are still unsatisfactory. To this end, we propose a relational reasoning network with parallel multi-scale attention for SOD in optical RSIs in this paper. The relational reasoning module that integrates the spatial and the channel dimensions is designed to infer the semantic relationship by utilizing high-level encoder features, thereby promoting the generation of more complete detection results. The parallel multi-scale attention module is proposed to effectively restore the detail information and address the scale variation of salient objects by using the low-level features refined by multi-scale attention. Extensive experiments on two datasets demonstrate that our proposed RRNet outperforms the existing state-of-the-art SOD competitors both qualitatively and quantitatively.
Pipeline
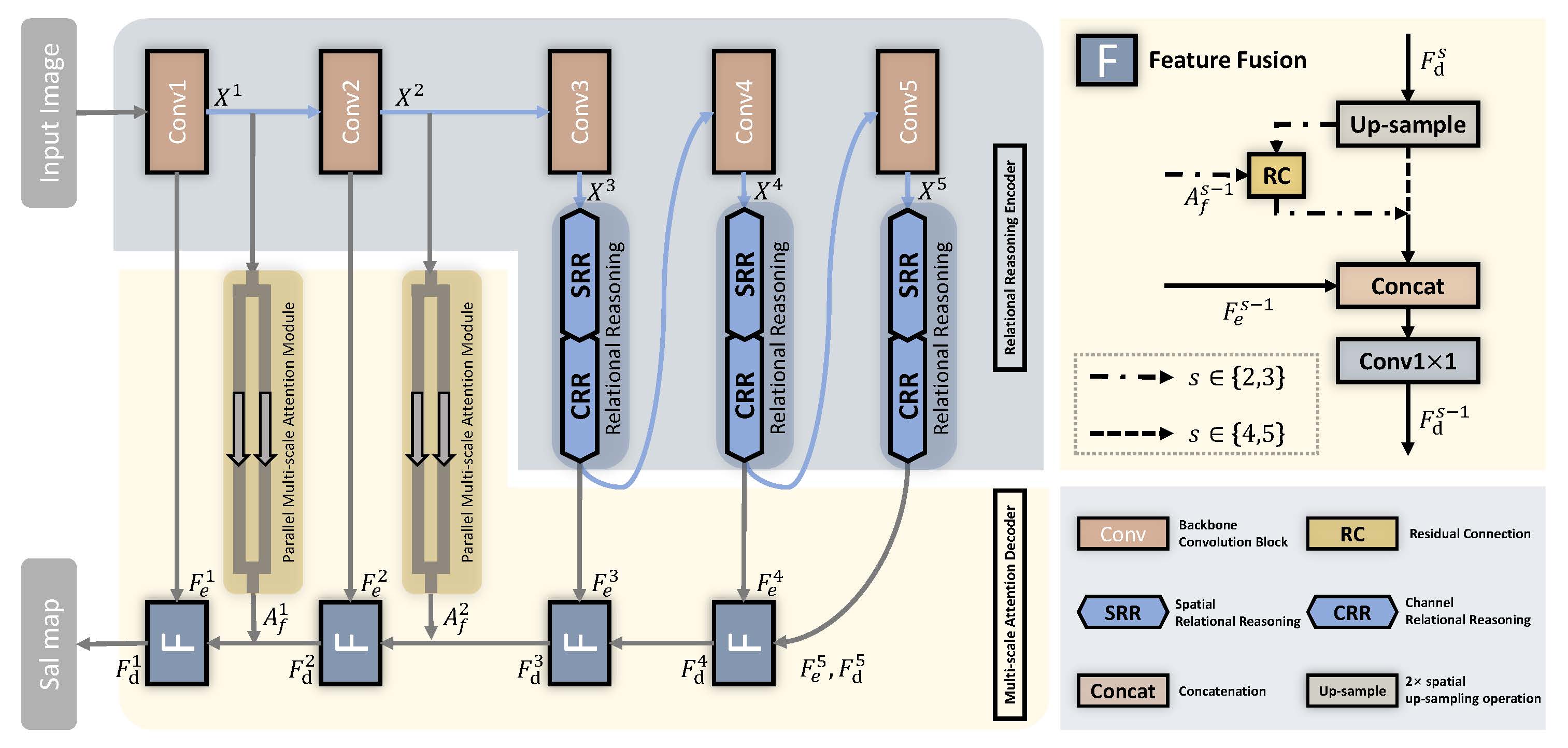
Architecture of RRNet, consisting of a relational reasoning encoder and a multi-scale attention decoder. The encoder generates hierarchical features, i.e., low-level features from the first two stages and high-level features from the last three stages. Relational reasoning in two dimensions following each high-level stage are successively employed to refine features by reasoning semantic relationship. Low-level features obtained by encoder are fed into parallel multi-scale attention module, generating attention maps with valuable information to restore lost details. The top right portion is the computation procedures of feature fusion between passed-up deep features and shallow features.
Highlights
We propose a novel end-to-end relational reasoning network with parallel multi-scale attention (RRNet) for SOD in optical RSIs, which consists of a relational reasoning encoder and a multi-scale attention decoder.
We design a relational reasoning module in the high-level layers of the encoder stage to model the sematic relations and force the generation of complete salient objects. This is the first attempt to introduce relational reasoning in the SOD framework for optical RSIs. Moreover, we innovatively employ relational reasoning along the spatial and channel dimensions jointly to obtain more comprehensive semantic relations.
We propose a parallel multi-scale attention scheme in the low-level layers of the decoder stage to recover the detail information in a multi-scale and attention manner. This mechanism can deal with the object scale variation issue through the multi-scale design, while effectively recovering the detail information with the help of shallower features selected by the parallel attention.
We compare the proposed methods with thirteen state-of-the-art approaches on two challenging optical RSI datasets. Without bells and whistles, our method achieves the best performance under three evaluation metrics. Besides, the model has a real-time inference speed of 109 FPS.
Qualitative Evaluation
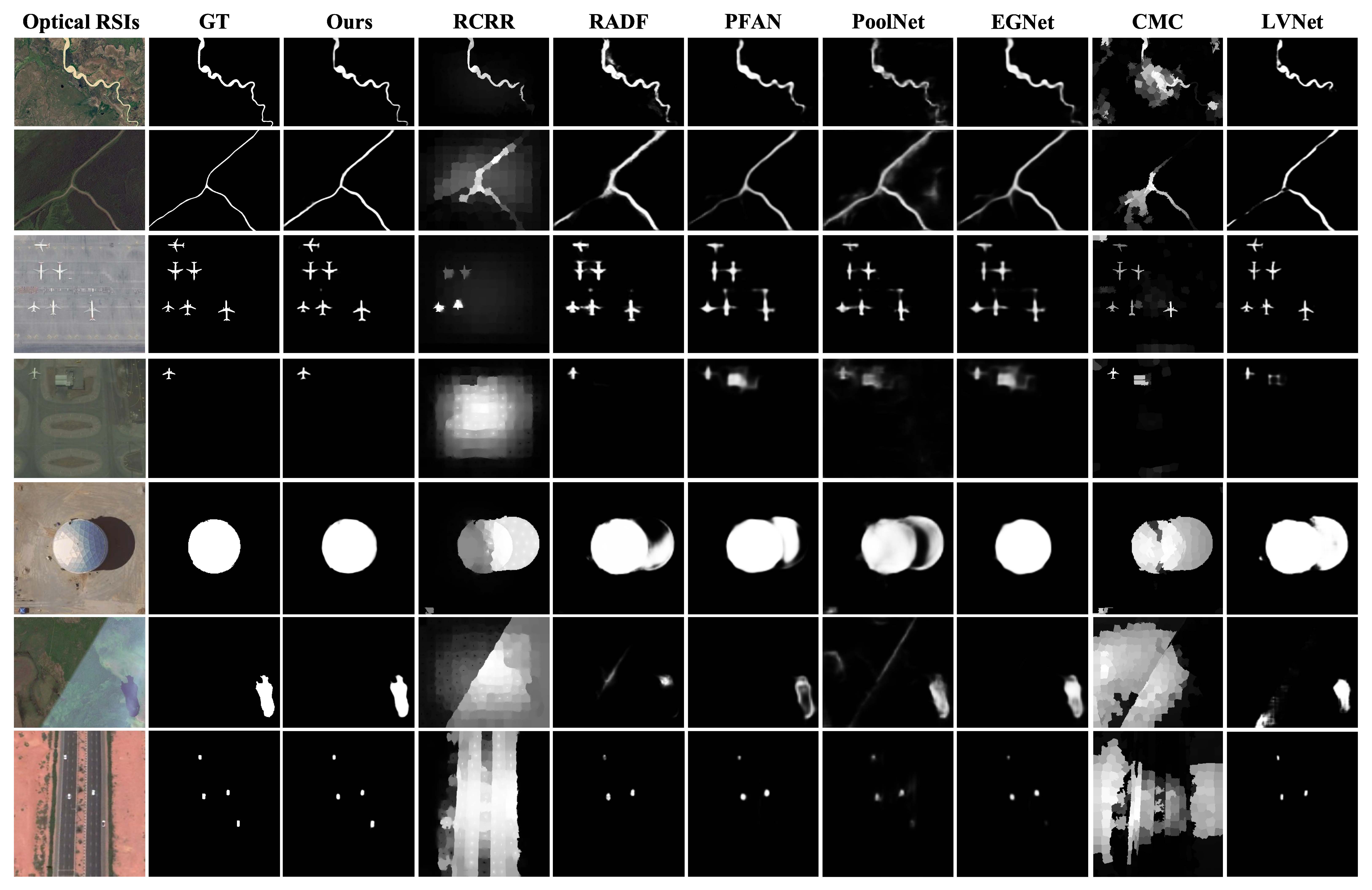
Visual comparisons of our proposed method and SOTA methods on EORSSD dataset. The deep learning based methods are trained/re-trained on the EORSSD dataset.
Quantitative Evaluation
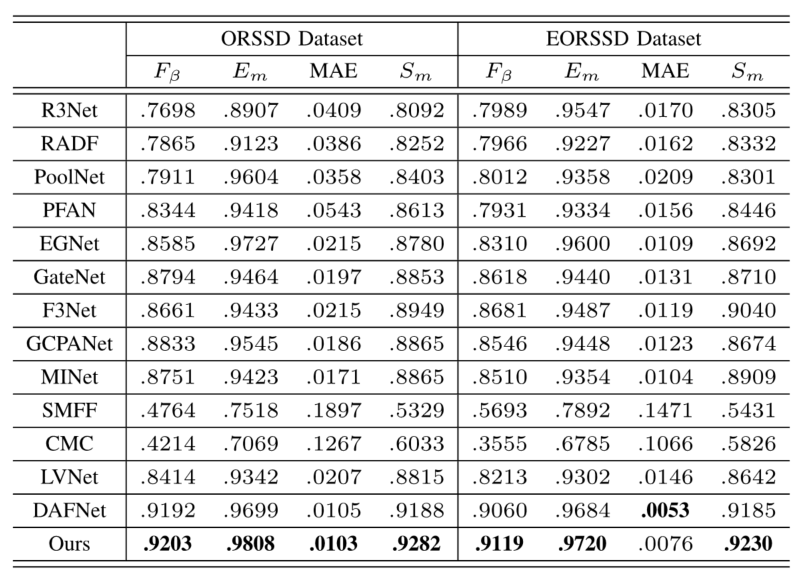
Other works
Qijian Zhang, Runmin Cong, Chongyi Li, Ming-Ming Cheng, Yuming Fang, Xiaochun Cao, Yao Zhao, and Sam Kwong, Dense attention fluid network for salient object detection in optical remote sensing images, IEEE Transactions on Image Processing, vol. 30, pp. 1305-1317, 2021. [Project Page]
Chongyi Li, Runmin Cong, Junhui Hou, Sanyi Zhang, Yue Qian, and Sam Kwong, Nested network with two-stream pyramid for salient object detection in optical remote sensing images, IEEE Transactions on Geoscience and Remote Sensing, vol. 57, no. 11, pp. 9156-9166, 2019. [Project Page]
Chongyi Li, Runmin Cong, Chunle Guo, Hua Li, Chunjie Zhang, Feng Zheng, and Yao Zhao, A parallel down-up fusion network for salient object detection in optical remote sensing images, Neurocomputing, vol. 415, pp. 411-420, 2020.
Citation
@article{RRNet, title={{RRNet}: Relational Reasoning Network with Parallel Multi-scale Attention for Salient Object Detection in Optical Remote Sensing Images}, author={Cong, Runmin and Zhang, Yumo and Fang, Leyuan and Li, Jun and Zhao, Yao and Kwong, Sam}, journal={IEEE Transactions on Geoscience and Remote Sensing}, volume={60}, pages={1558-1644}, year={2022}, publisher={IEEE} }
Contact
If you have any questions, please contact Runmin Cong at rmcong@bjtu.edu.cn.